Top Software Solutions for Effective Data Management
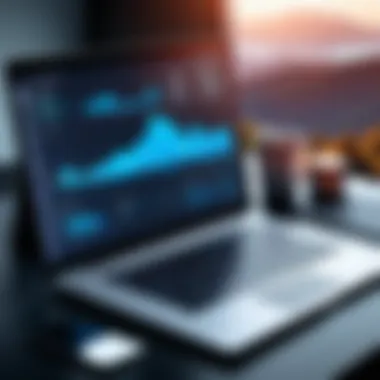
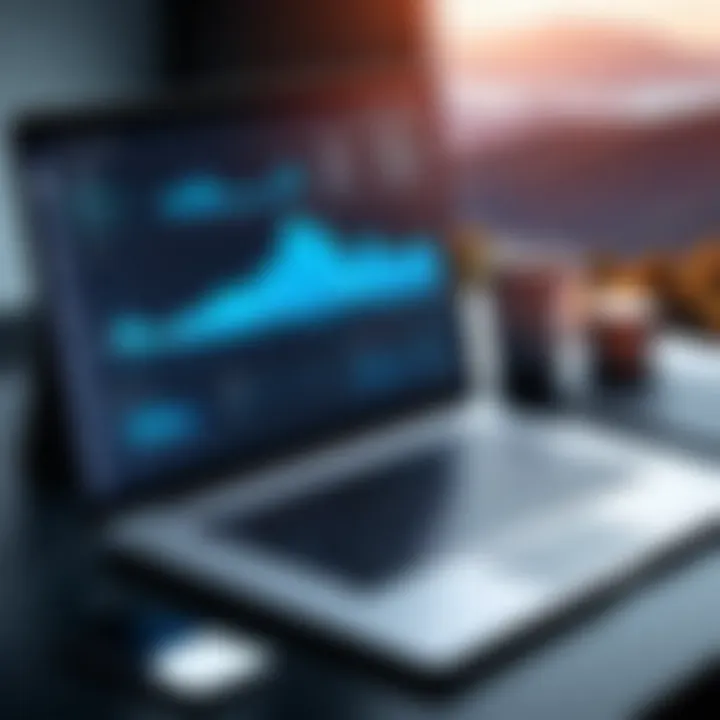
Intro
In today's business landscape, efficient data management is not merely a luxury, but a vital component for success. The rapid advancements in technology and the exponential growth of data means organizations need to harness the right tools for effective data handling. Choosing optimal software not only impacts immediate operations but also influences strategic decision-making and overall performance. This article will explore various software options, examining their unique features and practical applications to help you make an informed choice.
Software Overview
Software Description
Data management software envelops a range of tools designed to collect, organize, analyze, and share data. These solutions cater to different business sizes and industries, ranging from small startups to sprawling enterprises. Their primary goal is to transform raw data into meaningful insights while streamlining workflows and enhancing collaboration among teams.
Key Features
Effective data management software typically includes several key features:
- Data Integration: The ability to consolidate data from various sources, like internal databases or external APIs, ensures that you have a comprehensive view of your information.
- Collaboration Tools: Features like real-time editing and shared dashboards promote teamwork and improve decision-making.
- Data Security: With rising cybersecurity threats, robust encryption and access control measures are non-negotiable.
- Reporting and Analytics: Built-in tools for creating reports and visualizations can help in interpreting data at a glance. They often include customizable dashboards that provide the right information when needed.
- Scalability: As a business grows, its data needs will change. The right software should accommodate increasing datasets without losing efficiency.
"Data is a precious thing and will last longer than the systems themselves." – Tim Berners-Lee
Pricing Models
When investing in data management software, understanding the pricing structure is crucial. Here, we will break down the common pricing models that software providers offer.
Subscription Options
Most modern software solutions operate on a subscription basis. This approach allows businesses to pay a recurring fee to access the software, typically ranging from monthly to annually:
- Flexibility: Businesses can scale their subscriptions based on current needs, adjusting the number of users or features as required.
- Trial Periods: Often, vendors offer trial versions that enable users to test functionalities before committing financially.
One-Time Purchase
Some software can be purchased outright, where businesses pay a single fee to obtain a license. It can be a suitable choice for organizations that prefer a one-and-done model:
- Lower Long-Term Costs: While the upfront payment might be higher, this can save costs in the long run if regular updates or support are not required.
Selecting the right software for data management isn't just about budget, but about aligning a tool's capabilities with organizational needs. A deep understanding of both the software features and pricing models ensures better decision-making as you navigate the tools available in the market.
Understanding Data Software
In an age where information flows like a river, understanding data software becomes paramount for businesses that wish to navigate the waters of data management effectively. The right software solutions empower organizations to not just store data, but also analyze, retrieve, and utilize it in ways that drive decision-making and enhance operational efficiency. This section delves into the crucial aspects of data software, highlighting its definition, scope, and relevance in modern business environments.
Definition and Scope
Data software encompasses a broad category of tools and applications designed to gather, organize, store, and analyze data. This includes everything from standalone analytics platforms to comprehensive data management systems that integrate multiple functions. The scope of data software is vast, often overlapping with various technologies and methodologies aimed at improving data handling.
Such software is not a one-size-fits-all solution; instead, it caters to specific data-related tasks, ranging from data entry and extraction to intricate predictive analytics. Understanding these functionalities is vital for businesses seeking an edge in their competitive landscape. Each tool within the data software ecosystem serves a unique purpose, and organizations must determine which tools align with their specific needs.
Importance in Modern Businesses
For today’s enterprises, the importance of data software cannot be overstated. In a world increasingly driven by data, having the ability to leverage information effectively can spell the difference between success and failure. Here are a few reasons why data software is essential:
- Informed Decision-Making: With the right data tools, organizations can gather insights that inform strategy and operations. This capability enables businesses to make choices based on real data rather than gut feelings or outdated information.
- Operational Efficiency: Proper management software streamlines processes, reducing human error and saving time. This operational efficiency leads not only to increased productivity but often translates into reduced costs.
- Data Compliance and Security: In an era marked by stringent regulations regarding data privacy, having robust software solutions ensures businesses comply with existing laws and safeguard sensitive information.
"The key is in the details; understanding how to utilize data can turn what seems like chaos into clarity."
Types of Data Software
Understanding the various types of data software is a cornerstone of optimizing data management in any business. Each type serves a distinct purpose, offering unique functionalities that cater to different organizational needs. As businesses increasingly rely on data to drive decisions, the selection of appropriate software becomes critical in enhancing efficiency, ensuring accuracy, and enabling compliance.
When exploring data software, organizations should consider factors such as integration capabilities, user-friendliness, and scalability, as these aspects can drastically affect overall performance. These considerations are not merely technicalities but essential elements influencing a company’s success in a data-driven environment.
Data Analytics Software
Data analytics software plays a pivotal role in the decision-making process. It allows businesses to extract valuable insights from raw data, turning numbers into stories that inform strategy and action.
Key Features
One of the standout features of data analytics software is its ability to process vast amounts of data quickly and effectively. This processing power means that organizations can gain insights in real-time, which is a huge advantage in today’s fast-paced market.
For instance, tools like Tableau and Google Data Studio excel in visualizing data. Visualization makes complex data sets more understandable and facilitates quicker decision-making. However, the learning curve can sometimes be steep, particularly for users unfamiliar with data concepts. It requires investment in training to ensure teams can leverage these tools to their fullest potential.
Unique feature: Real-time data processing. Its advantage is that businesses can quickly adapt to new information; however, it requires strong computational resources and can be overwhelming for some users, especially in larger organizations where the data set complexity increases.
Use Cases
A major aspect of data analytics software is its versatility across different industries. For instance, in retail, businesses use such software to analyze customer behavior, which aids in inventory management and personalized marketing.
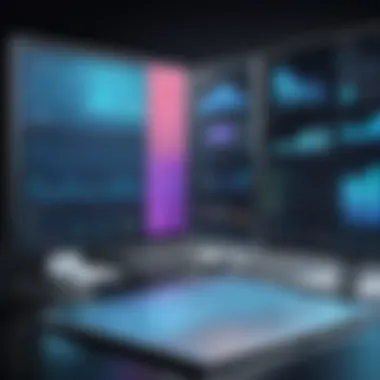
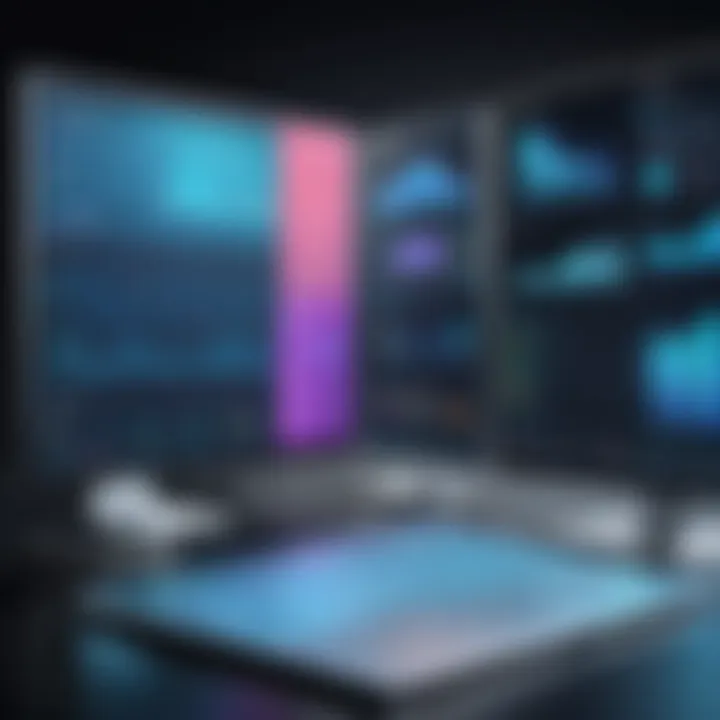
This adaptability makes it a popular choice among various sectors, underscoring its role in driving effectiveness and enhancing customer relationships. It is evident that, with appropriate context, data analytics can inform everything from product launches to marketing strategies, yielding significant competitive advantages.
Unique feature: Cross-industry applicability. The advantage of this versatility is that it can meet diverse needs; however, customization requires time and resources which might stretch smaller teams thin.
Data Management Platforms
Data management platforms (DMPs) are essential for integrating and managing data from multiple sources. With the growth of digital data, businesses need DMPs to gather, store, and curate information effectively.
Integration Capabilities
When considering integration capabilities, it's crucial to look at how well a DMP connects with existing systems in an organization. A robust DMP can pull data from various sources—like CRM, ERP, and social media.
This capability is beneficial because it centralizes data, offering a singular view for businesses to harness insights. However, poor integration is a common downfall, as system incompatibility can result in significant downtimes and frustrations.
Unique feature: Centralized data access. The advantage here is operational simplicity; however, if integration issues arise, they can lead to data silos, which contradict the very purpose of a DMP.
Data Governance
Another focal point in DMPs is data governance. Robust governance frameworks ensure that data is accurate, secure, and compliant with regulations. Given the increasing concern over data privacy, effective governance is paramount.
It can mitigate risks related to data breaches and non-compliance, providing peace of mind for businesses. But establishing these frameworks can be resource-intensive and requires a cultural shift in how organizations perceive and manage data.
Unique feature: Framework for compliance. The benefit is reduced risk of regulatory setbacks; however, smaller firms might find implementation challenging due to limited resources.
Business Intelligence Tools
Business intelligence tools are integral in converting raw data into actionable insights. These tools aid in reporting and enhance decision-making by helping businesses understand trends and patterns.
Visualization Features
One of the most significant aspects of BI tools is their visualization features. A well-designed tool can transform complex data sets into graphical representations that are easy to interpret.
This is especially important in communicating insights to stakeholders who may not be data-savvy. For instance, tools like Power BI and Looker are known for their user-friendly dashboards.
Unique feature: Interactive dashboards. This allows users to drill down into metrics, enhancing comprehension; however, an over-reliance on visuals can sometimes lead to oversimplification of data.
Reporting Functions
Reporting functions are another key element in BI tools. They facilitate the sharing of insights across the organization in a structured manner. These reporting capabilities ensure that decisions are data-driven and align with organizational goals.
However, the ability to generate customized reports often varies between tools, with some offering extensive customization—beneficial for detailed analysis—while others may be more rigid.
Unique feature: Custom reporting options. The advantage lies in tailoring reports to specific needs; yet, flexibility may come at the cost of simplicity, which can be daunting for novice users.
In sum, choosing the right type of data software is not just about picking a tool; it's about understanding its fit within your unique operational landscape. Therefore, a well-informed decision fueled by a comprehensive understanding of these types can lead a business toward meaningful advancement in data management.
Key Features to Consider
When delving into the realm of data software, the features available can make or break a user's experience. Choosing the right software isn't just about functionality; it's about how well these features align with the specific needs of the business. Thus, understanding the key features to consider is integral to making a well-informed decision.
User Interface and Experience
The user interface (UI) and overall user experience (UX) are paramount when selecting data management software. A good UI enables users to navigate the features and tools effortlessly. If the interface is clunky or counterintuitive, even the most powerful software can become frustrating. As technology evolves, companies must prioritize aesthetically pleasing and functional designs.
A clean workspace and straightforward dashboard can save time, making data management more efficient. Users may often find themselves juggling multiple tasks; thus, software that enhances productivity through its design can significantly impact day-to-day operations. Moreover, a positive UX encourages user adoption within teams, ensuring the software is utilized to its fullest potential.
Scalability
Scalability is another critical aspect when assessing data solutions. The right software must adapt to a company’s growth and evolving demands without requiring a complete overhaul. For instance, a small startup may start with basic features but later require advanced capabilities as it expands.
Opt for software that can grow with your organization. One day you might have ten users, and the next day, you'll need to accommodate hundreds. Solutions that allow for easy upgrades or additions to components can provide a smoother transition. In the end, the goal is to scale with efficiency and minimal disruption.
Compatibility with Existing Systems
In the age of technology, businesses often use a myriad of applications and systems to operate efficiently. Thus, ensuring that new data management software integrates smoothly with existing tools is crucial. Compatibility issues can lead to inefficiencies, additional costs, and user frustration.
A good practice is to conduct a thorough analysis of how the new solution will interface with current systems. Look for software with strong API capabilities or pre-built integrations, as they can facilitate smoother data flow. Whether you're using CRM systems, ERP platforms, or other specialized applications, seamless interoperability is essential for maintaining operational continuity.
Cost and ROI
When it comes to investing in software, cost is always a significant consideration. Evaluating the price against the potential return on investment (ROI) ensures a strategic acquisition. A higher price tag doesn’t necessarily equate to better quality; conversely, cheaper options might compromise essential features.
Therefore, it is prudent to assess the functions offered versus the price to ensure that the solution provides value. Factor in potential savings, increased efficiencies, and enhanced data management capabilities when estimating ROI. A well-chosen data management solution should be seen not just as an expense but an investment for the future of your organization.
"Software that aligns with business goals and user needs is not just a tool; it's a catalyst for success."
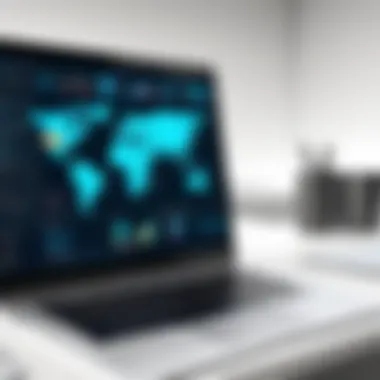
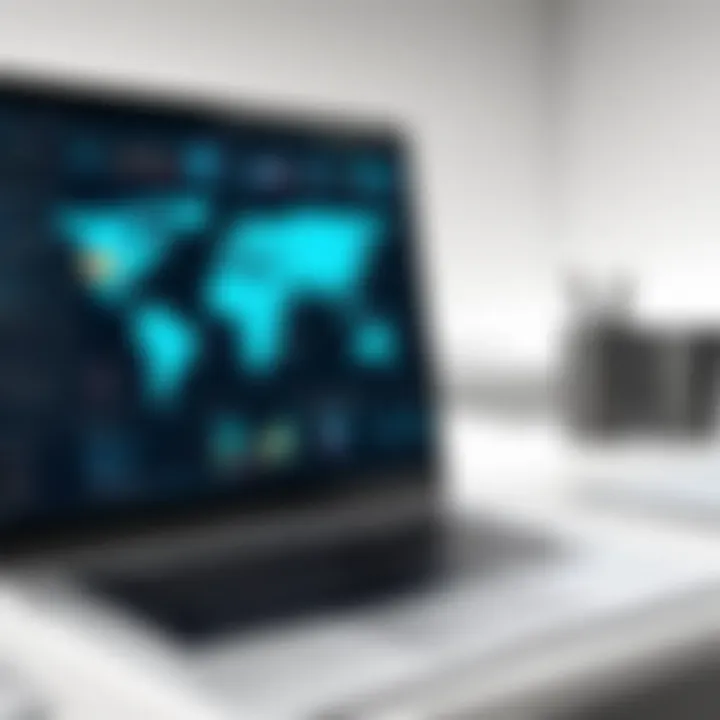
Understanding these key features is a fundamental step in discovering the most effective data management solutions. By prioritizing UI and UX, ensuring scalability, checking compatibility, and focusing on cost and ROI, organizations will be better equipped to harness the full potential of data software.
Top Data Software Solutions
When businesses think about how to manage their data efficiently, they’re often faced with a maze of choices. The importance of selecting the right Top Data Software Solutions can’t be overstated, since it directly impacts operational efficiency, decision-making processes, and even regulatory compliance. With data growing in volume and complexity, having a strong tool set that can handle this growth is not merely a luxury—it’s a necessity. Choosing the right data management software provides insights that can drive business strategies and help one stay ahead in competitive markets.
Comprehensive Overview
In exploring the landscape of data management, several standout software solutions emerge that cater to diverse business needs. These solutions often aim for streamlined operations while providing analytical capabilities that allow firms to slice and dice their data in meaningful ways. Popular choices among industry leaders include platforms like Tableau, Salesforce, and Microsoft Power BI. Each of these tools brings its unique strengths to the table, often padded with powerful features that can handle everything from real-time data processing to advanced analytics.
In essence, the best data software solutions strike a balance between ease of use and depth of functionality. They offer innovative features such as automated reporting, dashboard creation, and predictive analytics, making them conducive for both tech-savvy teams and those less inclined toward technology. This comprehensive suite enables better compliance with data governance practices, contributing to enhanced data accuracy and consistency across the board.
Comparative Analysis
Now let’s compare two heavyweights in this arena: Tableau and Power BI. Both software solutions are highly respected, yet they offer fundamentally different approaches.
Software A vs Software B
When it comes to Tableau vs Power BI, the contrast is quite telling. Tableau shines in its ability to create visually appealing, intricate data visualizations that make storytelling with data feel intuitive. Its user interface caters well to analysts and data scientists, enabling them to derive complex insights without the need for extensive coding. Moreover, what's noteworthy is its capacity for handling large data sets seamlessly.
On the other hand, Power BI is integrated deeply into Microsoft's ecosystem, making it a no-brainer for businesses using Microsoft products. Its ease of integration with tools like Excel and Azure enables quick deployment and a smoother learning curve for users already familiar with Microsoft environments. The key characteristic that makes it a popular choice is its affordable pricing model, which typically allows small to medium-sized enterprises to access robust features without breaking the bank.
Each software has its unique features that come with certain advantages and disadvantages. While Tableau focuses heavily on visuals and analytics depth, Power BI excels at producing quick reports and easy integrations. Understanding these nuances helps organizations choose the tool that fits their specific needs better.
Pricing Structures
Turning to Pricing Structures, this aspect is crucial and often serves as a deal-maker or breaker.
Tableau operates on a subscription basis, charging per user. This model can scale well, but can become costly as more users require access. Businesses must weigh the cost against the potential ROI brought on by enhanced capability for decision-making—this is often seen as a worthy trade-off for many organizations.
On the flip side, Power BI offers a more flexible pricing structure. It can provide a free tier for basic functionalities, which is an attractive option for startups and smaller businesses. The paid versions are also designed to cater to both individual users and teams, ensuring the software remains accessible. Still, there are instances where certain features might be restricted to higher tiers, which can limit smaller firms wishing to unlock advanced capabilities.
Evaluating User Feedback
Evaluating user feedback stands as a pillar in the realm of software solutions for data management. Understanding what actual users think about these tools offers a treasure trove of insights that can shape decision-making processes for organizations. User reviews act as a lens through which one can see the software’s performance in real-world scenarios, going beyond glossy marketing materials and promises.
Moreover, considering user feedback helps businesses identify potential pitfalls before diving headfirst into a new software initiative. When decision-makers understand how products resonate with their intended audience, they can tailor their choices to better suit their unique needs. Thus, keeping an ear to the ground and heeding the voices of current users is paramount for anyone looking to invest in data management software.
Importance of User Reviews
User reviews serve as critical indicators of a software's effectiveness and reliability. They provide a glimpse into how well a product meets expectations and handles tasks that matter most to users. Reviewing the experiences of previous customers can shed light on the usability of the software, its performance under load, and even customer support responsiveness. This information is invaluable in making informed choices.
Additionally, it’s often the case that the voice of the crowd speaks louder than any sales pitch. A product that shines in the eyes of its users can be a golden ticket for business owners and IT professionals alike. Conversely, glaring red flags in user feedback can steer others away from potential pitfalls, saving time and resources.
Patterns in User Experiences
Common Complaints
Common complaints frequently point out issues that might reappear across different users and environments. For example, many reviews on platforms like Reddit discuss poor integration capabilities with existing systems, a common headache for organizations migrating from older solutions. This insight can guide potential users to carefully assess integration tools available and ensure compatibility.
Such complaints can indicate underlying issues that need addressing, perhaps suggesting a less-than-stellar user experience design that frustrates users. Recognizing these patterns in complaints is crucial for businesses to understand what might impede their data flow or operational efficiency.
This segment of feedback is critical because it often highlights consequences that users have faced when implementing software in their day-to-day activities. It's not just a cry for help; it’s a message to future buyers about what could go wrong.
Favorite Features
On the flip side, identifying favorite features allows prospective users to gauge what makes certain software stand out. For instance, many users rave about the customizable dashboards that some software solutions offer. These features can significantly enhance user engagement, making the data more accessible and visually appealing to teams.
User favorites also often correlate with ease of use. As highlighted by reviews from various platforms, including Facebook groups centered on data management discussions, simplicity serves as a valuable asset that attracts robust user loyalty. When features are intuitive and powerful, they enhance overall productivity and make the software more enjoyable to work with.
In summary, evaluating user feedback is not merely about counting stars on a review site. It encompasses a rich landscape of user experiences that includes common complaints and favorite features, both of which provide a holistic view of what a software solution can deliver. This understanding can empower businesses to make more thoughtful, informed decisions as they navigate the complex world of data management.
Future Trends in Data Software
As we navigate the digital landscape, the importance of understanding future trends in data software cannot be overstated. This topic illuminates how organizations can better manage and utilize their data, ultimately steering their decision-making process and operational strategies. Recognizing these trends allows companies to stay ahead of the curve, ensuring they deploy software solutions that are not only effective but also adaptable to the swiftly changing environment. Let's dive into some of these transformative trends and their implications for businesses.
Emerging Technologies
Artificial Intelligence Integration
Artificial Intelligence (AI) integration plays a pivotal role in reshaping data management. One key characteristic of AI is its ability to analyze vast amounts of data with remarkable speed and accuracy. This capability enables businesses to glean insights that were previously difficult to uncover. For instance, through natural language processing, AI can sift through customer feedback, leading to improved products and services.
A distinct feature of AI in data software is predictive analytics. This allows companies to forecast trends and behaviors based on historical data, making it a popular choice among industry leaders. The benefits of incorporating AI include heightened efficiency and better strategic planning. However, there are challenges such as data privacy concerns and the need for significant investment in technology and training. Balancing these factors is crucial when considering AI integration.
Machine Learning Applications
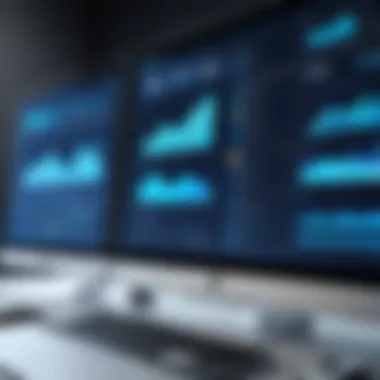
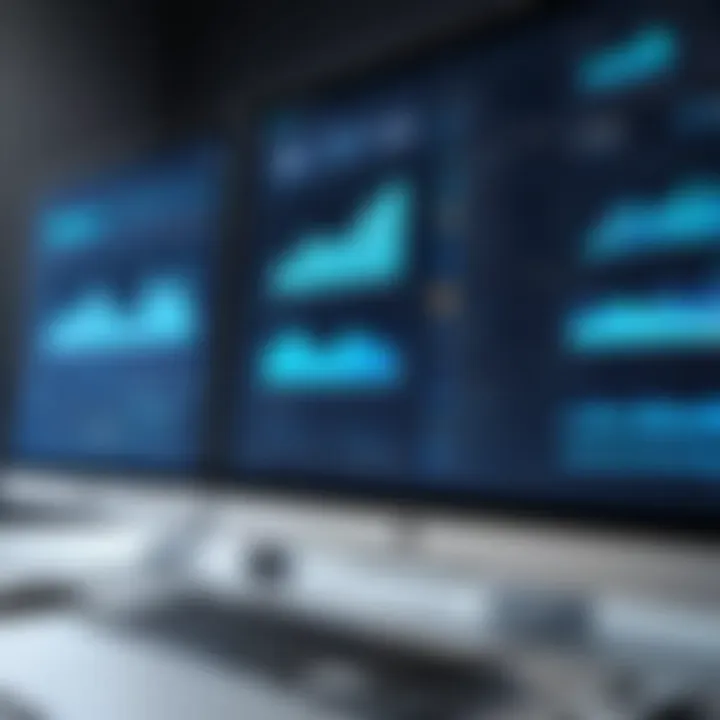
Machine Learning (ML) applications are another cornerstone of future data trends. They empower systems to learn from data inputs and progressively improve their performance without explicit programming. This self-improving capability offers businesses a chance to optimize their operations continuously.
A highlight of ML is its versatility; it can be applied across various sectors, from finance to healthcare, adapting to specific industry needs. This flexibility makes it a prevalent choice in data software solutions. Moreover, unique features such as anomaly detection allow firms to spot unusual patterns in data, aiding in fraud detection or identifying system errors early on.
While ML provides tremendous advantages, like enhanced accuracy and efficiency, it also comes with its share of pitfalls, like the potential for biased algorithms. Organizations must ensure they train their ML systems on diverse and representative datasets to mitigate these issues.
Shifts in Industry Standards
To keep pace with the evolving tech landscape, shifts in industry standards are increasingly evident. Organizations are transitioning to cloud-based data solutions, enhancing accessibility and collaboration. This shift not only helps in cutting costs but also allows for better scalability. Companies now need to prioritize security protocols in their data management strategies to comply with emerging regulations and protect sensitive information.
Challenges in Data Management
In any organization, data management is more than just a routine task; it's a critical function that can make or break a business. As we navigate through an ever-evolving digital landscape, companies face numerous hurdles. Understanding these challenges is vital for harnessing the full potential of data software solutions.
Data Security Concerns
Data security stands as one of the most pressing challenges organizations encounter. In an age where information is power, protecting sensitive data from breaches is paramount. With hackers constantly developing new techniques to infiltrate systems, companies need to be on their toes. A successful attack can lead to not just financial loss but also a severe dent to reputations that might take years to rebuild.
There are several key considerations that come into play:
- Sensitive Data Protection: Organizations must safeguard personal information, financial records, and proprietary data. For instance, implementing encryption and access controls is fundamental.
- Regular Audits: Conducting regular security assessments can help unearth vulnerabilities before they are exploited.
- Employee Training: Engaging employees in security awareness programs can significantly curb risks stemming from human error. Too often, a simple mistake leads to devastating breaches.
It’s not just about having the right software; it’s about fostering a culture of security mindfulness throughout the organization. By prioritizing data security, companies can build trust with their customers and partners.
"Data is the new oil. And like oil, it needs to be refined by those who can handle it carefully."
Compliance and Regulations
The regulatory landscape surrounding data management can feel like walking through a minefield. With laws such as GDPR in Europe and CCPA in California, businesses are under increasing pressure to comply with data governance policies. These regulations dictate how companies must handle personal data, requiring transparency and accountability.
There are several critical factors to consider:
- Understanding Local Regulations: Each state or region might have its own set of rules, and navigating through them is not for the faint of heart. Failing to comply can result in hefty fines or loss of business privileges.
- Implementing Proper Protocols: Companies must establish clear procedures for data collection, usage, and storage that align with these regulations. This often involves frequent data audits to ensure compliance is met at all times.
- Adaptability: As new regulations emerge, the ability to adapt quickly is essential. This requires continuous education and a willingness to evolve practices and technologies.
For many organizations, tackling compliance is part of a larger strategy to ensure long-term success and credibility in the marketplace. Ignoring these elements can do more than create operational issues; it can lead to legal ramifications that might haunt a business for years to come.
Overall, addressing these challenges in data management can not only safeguard a company’s assets but can also enhance its reputation and equip it for future growth. Understanding the nuances of data security and compliance lays the groundwork for leveraging data software in ways that contribute to robust business practices.
Implementation Considerations
When embarking on the journey to adopt data software solutions, the implementation stage can often prove to be a pivotal point in determining overall success. Many underestimate the complexity involved, but a careful approach to implementation can yield significant benefits down the line.
This section focuses on the crucial elements of initial setup, training, and support that organizations need to address to ensure that they maximize the value derived from their chosen data software tools. Here’s why paying meticulous attention to these facets makes a difference:
- Effective Use of Resources: A structured implementation plan helps allocate resources intelligently. Companies can avoid wastage of time and costs that come from haphazard efforts.
- Seamless Integration: Proper implementation ensures that new software blends smoothly with existing processes and technologies, minimizing disruptions.
- User Buy-In: Engaging users in the setup process fosters a sense of ownership. When employees feel involved, they are more likely to utilize the tools effectively.
"Software is only as good as how well it’s implemented and accepted by the team using it."
Initial Setup
Setting up data management software doesn't merely mean installing it on systems. It's a multi-step process that typically begins with thorough preparation. Businesses need to assess their specific needs and tailor the setup accordingly. Here's a clearer picture of what this encompasses:
- Needs Assessment: Understanding what you need is half the battle. This includes identifying which types of data you’ll manage, how it’ll be processed, and the scale of operations.
- Infrastructure Adjustments: Depending on the software chosen, organizations may need to upgrade or modify existing IT infrastructure. This could involve hardware changes or increases in cloud storage capabilities, ensuring compatibility with the new tools.
- Data Migration: Transitioning existing data into the new system requires accuracy and precision. Data cleansing should be part of the plan—removing duplicates and correcting errors can prevent future headaches.
- Setting User Roles: Deciding who gets access to what can often get overlooked. Clearly defining user roles at the outset ensures that sensitive data remains protected while empowering users with appropriate access.
Training and Support
Once the setup is complete, the next crucial element is training. Merely having sophisticated software isn't enough if users don't know how to engage with it. Effective training and ongoing support can significantly enhance user proficiency and confidence. Consider these aspects:
- User Training Programs: Organizations should invest in comprehensive training sessions tailored to different skill levels. While some employees may be tech-savvy, others might need more basic guidance. Interactive workshops, video tutorials, or one-on-one coaching can cater to diverse learning preferences.
- Documentation and Resources: Producing detailed manuals and easily accessible resources is essential. These should cover everything from installation down to advanced functionalities to guide users through various scenarios they may encounter.
- Feedback Mechanisms: Establishing lines of communication for users to provide feedback on training can help adapt the program for future needs. Continuous improvement must be part of the culture.
- Ongoing Support: Post-implementation, businesses should offer continuous support. This might involve a dedicated help desk, regular check-ins, or online forums to troubleshoot and discuss challenges. Having reliable support can minimize frustrations and enhance user satisfaction, ensuring that the software's full potential is tapped.
Measuring Success Post-Implementation
Understanding how to measure success after implementing data management software is crucial for any organization. It’s not just about making the switch but knowing what comes after. This stage is vital for validating that the selection of software was appropriately aligned with business goals. Organizations pour resources into these tools, and tracking progress post-implementation ensures that the investments yield some form of return and support better decision-making in future endeavors.
Performance Metrics
Identifying the right performance metrics is like hitting the nail on the head. These indicators offer quantifiable evidence of how well the data management software is performing against the initial goals set out by the organization. Here are a few key metrics often considered:
- Data Accuracy: Measures how well the software maintains accurate data over time. A good score here reflects that the data being worked with is reliable.
- User Adoption Rate: This tracks the percentage of employees actively using the new software. High adoption indicates that the software is deemed useful by the team.
- Processing Time: Evaluating how long it takes to retrieve and analyze data post-implementation gives insight into efficiency improvements.
- Cost Savings: Assess whether operational costs have decreased, which can be tied directly to the effectiveness of the software in streamlining tasks.
For instance, if an organization was struggling with slow data access, and after implementation sees a reduction from several hours to mere minutes, that's a clear indication of improvement. These metrics, when monitored regularly, can guide adjustments necessary to align ongoing activities with corporate objectives.
Continuous Improvement Strategies
After establishing success metrics, it’s time to pivot towards continuous improvement strategies. The digital landscape is dynamic, and simply having top-tier software today doesn’t guarantee seamless operation tomorrow. Thus, maintaining a routine for examination and enhancement is essential. Some strategies include:
- Regular Training Sessions: Hosting ongoing training helps keep the employees’ skill set sharp and ensures they're aware of new features and updates in the software.
- Feedback Mechanisms: Encouraging user feedback facilitates a direct line of communication about what is working well and what’s not. It allows for real-world insights into potential improvements.
- Quarterly Reviews: Conducting reviews periodically can show shifts in performance metrics over time. Look for patterns that hint at decay or growth in software effectiveness.
To bolster the notion of continuous improvement, consider implementing an agile approach. Instead of waiting for the annual review cycle, fostering a culture of incremental progress can yield faster adjustments. Every little tweak can pile up, leading to massive improvements down the line.
"It's not the tools themselves that define success, but how organizations adapt their strategies around them and commit to ongoing learning and enhancements."
Ultimately, the goal of measuring success post-implementation isn’t just for the sake of accountability; it’s about setting organizations on a path toward sustainable growth and adaptability in an ever-evolving data landscape.