Clinical Conversational AI: Revolutionizing Healthcare Communication
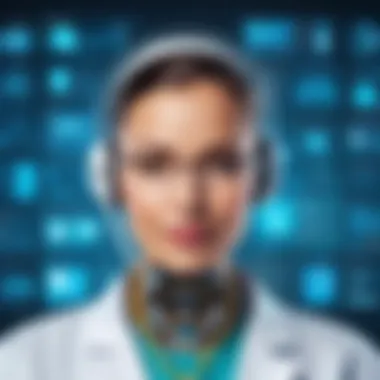
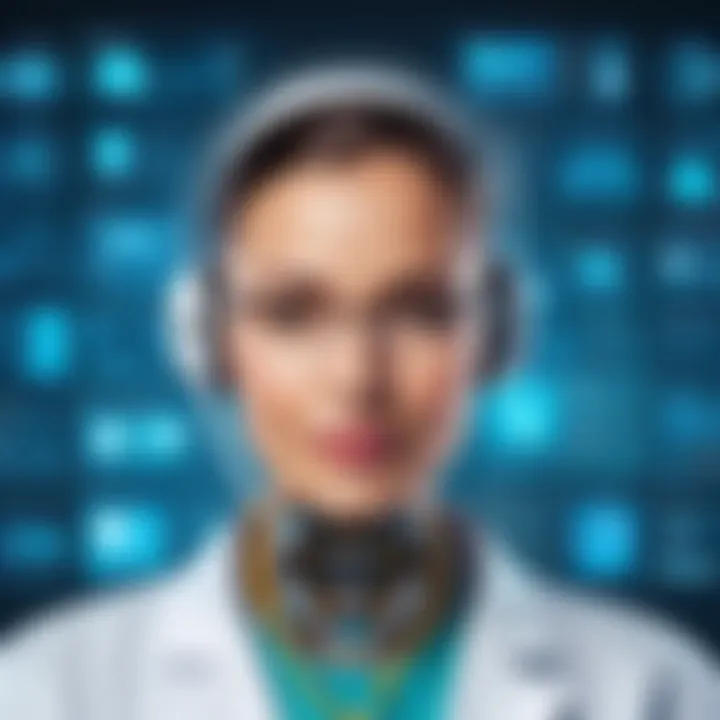
Intro
The integration of conversational AI technologies in clinical settings marks a significant evolution in healthcare communication. With advancements in artificial intelligence, healthcare providers can now leverage these tools to enhance patient interactions, improve clinical workflows, and facilitate accurate data management. As healthcare continues to embrace digital transformation, understanding the implications of clinical conversational AI becomes essential for stakeholders, including IT professionals and decision-makers.
This article will dissect various facets of clinical conversational AI, providing insight into its software foundations, key features, and the specific benefits as well as challenges faced in its implementation. The aim is not only to highlight the technical aspects but also to emphasize the broader impact on patient engagement and healthcare delivery. By exploring the technology's capabilities and real-world applications, we can better appreciate its role in reshaping how healthcare communication unfolds.
The sections that follow will guide readers through the intricate landscape of clinical conversational AI, offering a detailed resource tailored to professionals engaged in optimizing healthcare technologies.
Software Overview
Software Description
Clinical conversational AI refers to AI-powered systems designed to simulate human-like interactions with patients and healthcare personnel. These systems can include chatbots, voice assistants, and other digital platforms that facilitate communication within clinical environments. By utilizing natural language processing and machine learning techniques, these systems can comprehend and respond to patient inquiries, streamline appointments, and even assist in chronic disease management.
A few notable applications include virtual appointment schedulers, symptom checkers, and post-visit follow-up tools. Each application serves distinct purposes, contributing to a comprehensive healthcare communication strategy.
Key Features
Effective clinical conversational AI software often includes several critical features, enhancing its usability and functionality:
- Natural Language Understanding (NLU): This allows the AI to comprehend and process human language effectively, increasing the accuracy of interactions.
- Integration Capabilities: These systems typically need to integrate seamlessly with existing electronic health records (EHR) and other healthcare management systems to ensure smooth data flow.
- Scalability: As healthcare demands grow, the AI should accommodate an increasing volume of patient interactions without a loss in quality.
- Data Security: Ensuring patient information remains confidential is paramount. Robust security protocols must be integrated into the software design.
- User-Friendly Interface: A clear and easy-to-use interface encourages adoption among both healthcare professionals and patients, fostering better communication.
"The adoption of conversational AI technology in healthcare not only improves patient experience but also optimizes clinical operations, making for a more streamlined and efficient healthcare environment."
By recognizing these features, healthcare providers can better evaluate the tools they consider deploying within their organizations, ensuring alignment with operational goals and patient needs.
Understanding Clinical Conversational AI
Clinical conversational AI is becoming increasingly relevant in the healthcare landscape. This technology serves to streamline communication between patients and healthcare providers. Understanding it goes beyond just acknowledging its existence; it involves exploring its functionalities and potential benefits for health systems. The insights gained here help in recognizing how communications can be radically transformed, thus enhancing patient engagement and operational efficiencies.
Definition and Scope
Clinical conversational AI refers to software systems designed to understand and generate human language. Its scope encompasses various technologies such as natural language processing and machine learning. These systems can engage in dialogue with users, often simulating human conversations. This interaction is crucial in a healthcare setting where accurate information exchange can significantly impact patient outcomes.
The scope also includes assessment and management of patient inquiries, data management, appointment scheduling, and more. Conversational AI can act as a supportive tool to healthcare providers. It allows for faster resolution of queries. This technology can be used across multiple platforms such as websites, mobile applications, and even patient kiosks in hospitals.
Historical Context and Evolution
The journey of clinical conversational AI is marked by significant milestones. Originally, artificial intelligence was primarily focused on data processing and rudimentary task automation. However, the push towards more human-like interaction brought forth advancements in language understanding and machine learning.
In the early days, AI in healthcare revolved mainly around simple decision support systems. As technology progressed, more sophisticated systems began to emerge. The integration of natural language processing by companies like IBM, with its Watson system, was a key turning point. This advancement enabled deeper conversational capabilities and brought AI closer to real-world applications.
Over the years, clinical conversational AI has evolved from basic chatbots to sophisticated systems capable of multi-turn dialogues with contextual understanding. Now, organizations are considering these AI tools to not only enhance efficiency but also to provide tailored patient experiences. The historical development illustrates the fruitful intersection of technology and healthcare communication. It signals a significant change in how information is conveyed and processed within clinical environments.
Technological Framework
The technological framework is crucial to understanding the workings of clinical conversational AI. It encompasses the essential technologies that enable these systems to process human language, learn from interactions, and integrate into existing healthcare environments. This section highlights key elements, benefits, and various considerations surrounding this framework, ultimately shaping the efficacy of conversational systems in healthcare communication.
Natural Language Processing Essentials
Natural language processing (NLP) is at the heart of conversational AI. It allows machines to understand, interpret, and respond to human language in a way that makes sense. In healthcare, NLP is significant for several reasons:
- Patient Query Handling: NLP can efficiently process patient inquiries, making it easier to extract relevant information and respond appropriately.
- Sentiment Analysis: This helps gauge patient emotions. Understanding a patient's feelings can enhance care quality.
- Data Extraction: NLP can sift through unstructured data, enabling healthcare providers to extract essential information from patient records.
These capabilities make NLP a vital component of conversational AI, ensuring it can interact with patients effectively and understand medical context.
Machine Learning Techniques in Conversational AI
Machine learning (ML) techniques are integral to improving how conversational AI functions over time. Through various algorithms, these systems learn from past interactions, adapting their responses and enhancing their accuracy. Key techniques include:
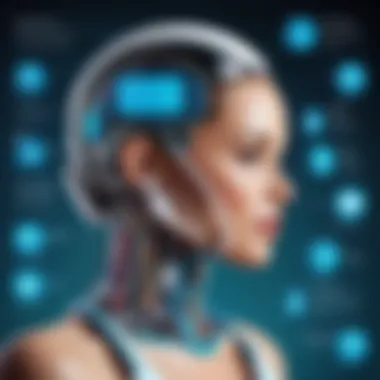
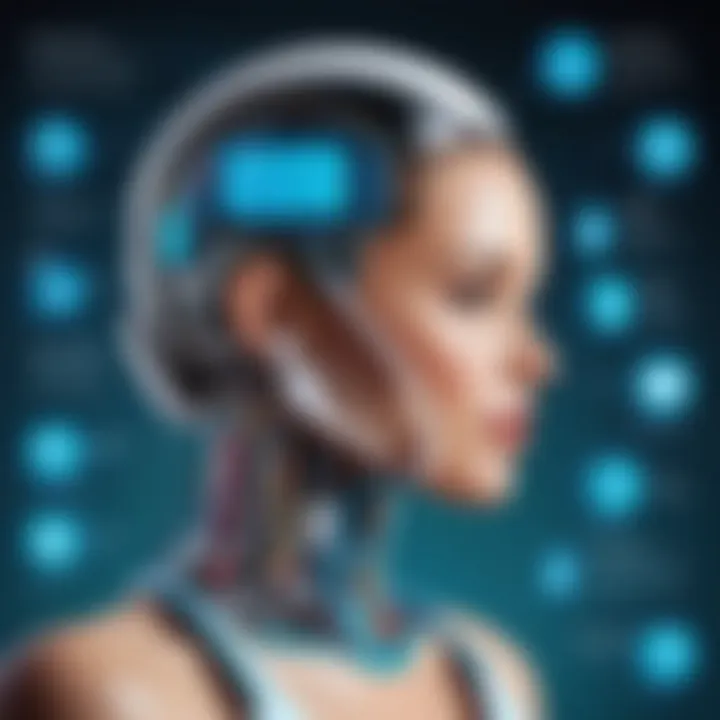
- Supervised Learning: It involves training a model on labeled data. This is useful in medical settings for precise classification of symptoms from patient inputs.
- Unsupervised Learning: Here, the model identifies patterns without labeled data. It can be beneficial in discovering trends in patient inquiries.
- Reinforcement Learning: This method uses feedback from interactions to improve future responses. In a clinical setting, the AI system becomes more efficient over time, requiring less human intervention.
Utilizing these techniques not only increases efficiency but also fosters a more interactive patient experience.
Integration with Existing Healthcare Systems
Integrating conversational AI systems with current healthcare infrastructures is a complex yet rewarding endeavor. The importance of seamless integration cannot be overstated. It supports:
- Data Consistency: By connecting with existing systems, AI can access consistent datasets, which aids in accurate information retrieval and decision-making.
- User Experience: Patients benefit significantly when conversational systems work within pre-existing platforms, allowing for smoother interactions.
- Cost Efficiency: Integration can reduce operational costs, as it minimizes the need for additional interfaces or data silos.
While integration is essential, it does present challenges such as data privacy concerns and potential technical barriers. However, with thoughtful design and implementation, these hurdles can be overcome.
"The backbone of effective conversational AI in healthcare lies in its technological framework—essential for enhancing communication and patient engagement."
Applications of Conversational AI in Healthcare
The application of conversational AI in healthcare represents a significant stride towards improving communication, efficiency, and overall patient experience. This section delves into specific areas where conversational AI has made a tangible impact, enhancing both patient interactions and administrative processes. Understanding these applications is crucial for healthcare professionals as they work to integrate advanced technologies into their practices.
Patient Engagement and Support
Patient engagement is a central focus in modern healthcare. Conversational AI provides an avenue for developing personalized interactions that encourage patients to take charge of their health. For example, AI-driven chatbots can facilitate 24/7 communication, answering questions, managing appointment schedules, or reminding patients about medication. This constant interaction not only improves the availability of care but also helps in building rapport between patients and healthcare providers.
Moreover, conversational AI can streamline the onboarding process for new patients. By using tailored scripts based on patient information, machines can guide users through registration or initial assessments. This practice reduces waiting times and enhances data collection accuracy.
Patients often appreciate having immediate answers without waiting for human involvement. The result is a potential increase in adherence to treatment plans and greater satisfaction with the care process.
Administrative Functions
Beyond direct patient support, conversational AI plays an indispensable role in administrative functions within healthcare settings. The integration of AI can automate repetitive tasks that consume a significant amount of time for staff. Tasks such as scheduling, billing inquiries, and insurance verifications can be managed by AI systems, freeing up staff to focus on more critical aspects of care.
This automation can lead to reduced operational costs. For instance, hospitals such as Mount Sinai have implemented chatbots for managing appointment bookings, which has resulted in a notable decline in no-shows and cancellations. These systems help to optimize resource allocation, ensuring that healthcare providers can serve more patients without compromising service quality.
Enhanced Medical Diagnostics
Conversational AI can also aid in enhanced medical diagnostics by supporting clinical decision-making. AI tools analyze patient data and guide healthcare professionals with pertinent information during consultations. For example, systems can ask patients preliminary questions, analyze their inputs, and provide insights before the healthcare provider engages directly. This method helps ensure that the clinician is better prepared with relevant data, making the diagnostic process more efficient.
Such AI systems can identify potential health issues before a consultation, prompting further investigation and faster interventions. This capability is particularly valuable in cases where early detection leads to improved patient outcomes. It also contributes to a more informed and efficient use of consultation time.
"Incorporating conversational AI in healthcare applications does not merely streamline processes; it reshapes the entire patient experience by enhancing interaction quality and operational efficiency."
In summary, the applications of conversational AI in healthcare reveal its transformative potential. From augmenting patient engagement to optimizing administrative tasks and enhancing diagnostics, these technologies are poised to redefine how healthcare providers operate and interact with those they serve.
Benefits of Clinical Conversational AI
The significance of clinical conversational AI in healthcare communication is profound. By enhancing interaction between patients and professionals, this technology serves to streamline workflows and improve outcomes. The integration of AI generates multiple benefits that address some of the longstanding challenges faced in clinical environments. The following subsections illustrate these benefits in detail, providing a clearer picture of how conversational AI can shape the future of healthcare.
Improved Efficiency in Communication
One of the primary advantages of clinical conversational AI is the substantial improvement in communication efficiency. Traditional methods of patient interaction often suffer from delays and miscommunications. With conversational AI, the process of information exchange becomes more fluid and responsive. AI systems can manage numerous inquiries simultaneously, thus alleviating the burden on healthcare staff. For instance, chatbot systems such as Ada Health enable patients to receive immediate responses to their questions, significantly decreasing wait times. This enhancement allows medical professionals to dedicate more time to critical tasks rather than repetitive inquiries.
Furthermore, automated systems can be available 24/7, permitting patients to access information outside standard hours. This round-the-clock availability is essential, especially in situations where timely responses are crucial.
"AI in healthcare enhances not just communication but operational productivity across various fronts."
Enhanced Patient Satisfaction
Another critical benefit of clinical conversational AI is the increase in patient satisfaction. Engaged patients are more likely to adhere to medical advice and follow-ups. Conversational AI systems focus on personalized communication strategies that cater to individual needs. Such an approach can foster a sense of understanding and empathy, thereby enhancing the overall patient experience.
For example, applications like Buoy Health utilize conversational AI to guide patients through symptom assessment while providing tailored health recommendations. This not only empowers patients with knowledge but also ensures they feel heard and valued. Satisfied patients are more likely to recommend services and contribute positively to the healthcare entity’s reputation.
Data Collection and Analysis Capabilities
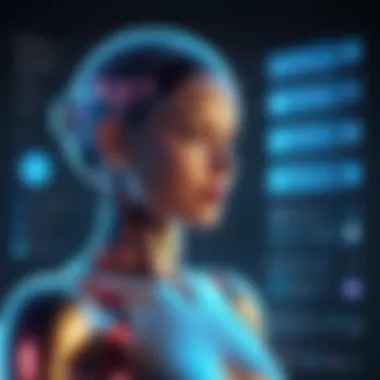
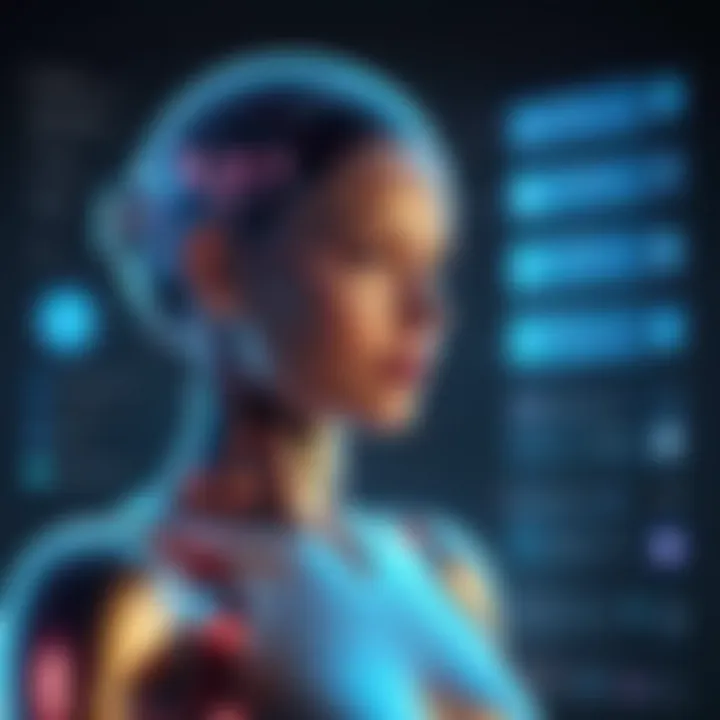
Data handling is an integral aspect of healthcare diagnostics and management. Clinical conversational AI enhances these capabilities through seamless data collection and analysis. AI systems effectively gather and process data points during interactions, contributing to richer datasets that can be analyzed for insights.
With tools like IBM Watson Health, health professionals can harness AI’s analytical power to make informed decisions based on real-time data gathered through patient interactions. By identifying trends and patient needs, healthcare providers can improve service quality and enhance preventive measures.
In summary, the benefits of clinical conversational AI extend beyond superficial enhancements. They create a more effective and responsive healthcare environment. With improved efficiency in communication, enhanced patient satisfaction, and powerful data analysis capabilities, clinical conversational AI positions itself as a cornerstone in modern medical practice. As the field continues to evolve, understanding these benefits becomes essential for stakeholders aiming to improve healthcare delivery systematically.
Challenges and Limitations
The integration of clinical conversational AI into healthcare is not devoid of challenges. Understanding these challenges is vital for successful implementation. This section discusses data privacy concerns, technological barriers, and limitations in human-like interaction, all of which can influence the efficacy of conversational AI systems in the healthcare sector.
Data Privacy Concerns
One of the most pressing issues surrounding the use of conversational AI in clinical settings is data privacy. Patient data is highly sensitive and governed by strict regulations, such as the Health Insurance Portability and Accountability Act (HIPAA) in the United States. Ensuring compliance with these regulations is paramount.
Conversational AI systems process vast amounts of data, requiring healthcare providers to implement robust security measures. Failure to protect this data not only jeopardizes patient confidentiality but also exposes healthcare organizations to legal ramifications. Data breaches can result in significant financial penalties and damage to reputation.
Organizations must prioritize data encryption, access controls, and regular audits to safeguard patient information while using conversational AI. Moreover, transparent communication with patients about how their data is used can enhance trust and ease concerns.
Technological Barriers to Adoption
Despite the potential of conversational AI, several technological barriers hinder its widespread adoption in healthcare. Many healthcare facilities operate on legacy systems that are not compatible with modern AI technologies. Integrating new systems may require significant investment in upgrades or replacements.
Additionally, the development and maintenance of conversational AI systems demand substantial technical expertise. Many healthcare organizations lack the necessary personnel skilled in AI and machine learning, presenting a barrier to implementation. Consequently, training existing staff or hiring specialists is crucial, but it also adds to operational costs.
Interoperability between various platforms is another challenge. Many AI systems need to seamlessly connect with electronic health records and other management systems to be effective. A lack of standardized protocols can create inefficiencies and complications in data exchange.
Limitations in Human-Like Interaction
Although conversational AI has advanced significantly, it still faces limitations in replicating human-like interaction. Effective communication is essential in healthcare, where empathy and understanding play vital roles in patient care. Current AI systems struggle to grasp nuanced emotions and context in conversations, resulting in suboptimal interactions.
Consequently, patients may find AI systems impersonal, which can diminish their experience. For instance, chatbot responses may lack empathy or fail to address patients' specific concerns adequately. This limitation can lead to frustration among users and may reduce overall engagement with the technology.
Moreover, while machine learning algorithms can improve over time, they still require vast amounts of data to ensure accuracy in responses. Relying solely on AI for patient interactions may limit the comprehensiveness of care provided.
The potential of AI in healthcare is immense, yet the challenges presented must be systematically addressed for successful implementation.
Evaluating these challenges and limitations is crucial for any organization considering adopting clinical conversational AI. By addressing data privacy, overcoming technological barriers, and improving human-like interaction, healthcare entities can optimize the integration of conversational AI, ultimately enhancing patient care and operational efficiency.
Evaluating Conversational AI Systems
Evaluating conversational AI systems is a critical part of integrating these technologies into healthcare settings. The implications of these evaluations are numerous and complex. Proper evaluation not only gauges the effectiveness of these tools but also ensures that they fulfill their intended purpose in patient engagement, administrative processes, and diagnostics.
Through effective evaluation, healthcare professionals can determine how well these systems perform, understand their limitations, and identify areas for improvement. This, in turn, enhances decision-making processes and promotes transparency when using AI in clinical settings.
Key Performance Indicators
Key performance indicators (KPIs) are essential metrics used to measure the effectiveness of conversational AI systems. These indicators provide a clear picture of how well these technologies perform in various scenarios. Common KPIs include:
- Accuracy of Responses: How often does the AI provide correct answers to patient inquiries?
- Customer Satisfaction Rates: What percentage of users report a positive experience when interacting with the system?
- Response Times: How quickly does the AI respond to user queries?
- Engagement Levels: Are users interacting with the AI as intended, or is there a drop-off in engagement?
- Error Rates: How often do misunderstandings or inaccuracies occur during interactions?
Monitoring these KPIs is vital to understanding how conversational AI can better support healthcare communication. Analyzing these metrics helps organizations to make informed decisions about technology upgrades, staffing needs, and the potential for expanded functionalities.
User Testimonies and Case Studies
User testimonies and case studies offer valuable insights into the real-world application of conversational AI systems. They provide a narrative that transcends mere statistics, highlighting personal experiences and outcomes that quantitative data alone cannot convey.
For instance, many healthcare providers have reported significant improvements in patient satisfaction following the implementation of conversational AI tools. One prominent case study involved the use of the chatbot, Ada Health. Patients using Ada reported faster response times for their medical inquiries, leading to better symptom management.
Furthermore, testimonies from healthcare staff often reveal how these systems free them from repetitive tasks. This allows providers to focus on more critical aspects of patient care, illuminating a hidden benefit of integrating AI into clinical workflows.
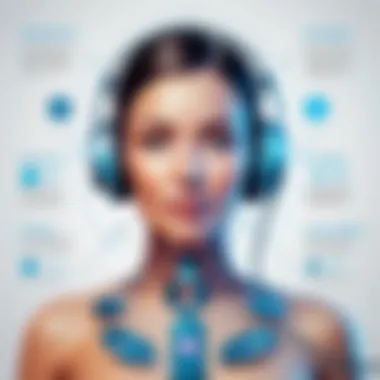
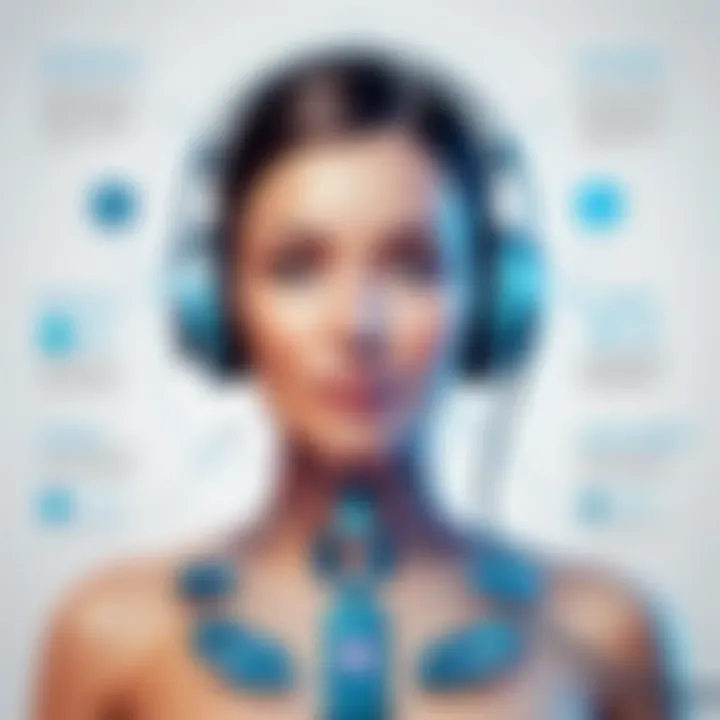
"Implementing conversational AI has transformed our approach to patient engagement and reduced response times significantly," noted a healthcare administrator at a prominent hospital.
In summary, evaluating conversational AI systems through KPIs, user testimonies, and case studies can paint a holistic picture of their effectiveness and impact on healthcare communication. Continuous assessment is essential to refine these systems, ensuring they meet the evolving needs of patients and providers alike.
Regulatory and Ethical Considerations
The integration of clinical conversational AI into healthcare settings invites scrutiny not only from a technological standpoint but also in terms of regulations and ethics. As AI systems become more prevalent, ensuring compliance with established healthcare regulations is crucial. This compliance helps to protect sensitive patient information and maintains the integrity of healthcare practices. Furthermore, understanding the ethical implications of deploying AI in patient care fosters trust among healthcare providers and patients alike.
Compliance with Healthcare Regulations
Compliance with healthcare regulations involves adhering to laws and standards that govern the practice of medicine. These regulations include HIPAA in the United States, which mandates the protection of patient data, as well as various global frameworks aimed at securing personal health information.
A key element of compliance is the implementation and deployment of AI systems that will handle patient interactions. Organizations need to conduct thorough risk assessments to identify areas of potential data breaches and establish safeguards to mitigate these risks. A clear understanding of regulatory frameworks not only ensures legal compliance but also enhances the credibility of the technologies being utilized.
Moreover, organizations must prioritize transparency in their conversational AI systems. Transparency involves making sure that patients are aware of how their data is being used and stored. This openness aids in building trust and rapport between patients and healthcare providers, which is essential for patient adherence to treatment plans and clinical recommendations.
Ethical Implications of AI in Patient Care
The ethical landscape of integrating AI into patient care presents numerous challenges and considerations. One significant concern is the issue of informed consent. Patients must be fully informed about the role of AI in their care, including its capabilities and the limitations inherent in the technology. This understanding is important so that patients can make informed choices and are aware of any potential risks associated with AI-driven recommendations.
Additionally, the potential for bias in AI algorithms poses a critical ethical challenge. If AI systems are trained on datasets that lack diversity, they may perpetuate stereotypes or provide unequal care to different demographic groups. This is where the role of stakeholders in overseeing AI development is vital. Continuous evaluation and adjustment of AI systems must happen to ensure equity and fairness in healthcare delivery.
"Ethics in AI is not just about making decisions, it’s about making the right decisions that benefit all stakeholders involved."
Lastly, the dependence on AI tools raises questions about accountability. In scenarios where an AI system makes a critical decision regarding patient care, it is unclear who should be held accountable if that decision leads to adverse outcomes. Establishing clear guidelines regarding accountability is crucial for ensuring a responsible approach to deploying AI in healthcare.
The Future of Clinical Conversational AI
The future of clinical conversational AI is an area of great significance for healthcare communication. As technology continues to evolve, so too does the potential for conversational AI to improve efficiency, broaden accessibility, and enhance the overall quality of patient care. This section provides insights into emerging trends and prospective innovations that shape the future landscape of clinical conversational AI.
Emerging Trends
Several trends are bolstering the trajectory of conversational AI in the healthcare sector. First, personalized patient experiences are becoming a focal point. AI technology is being used to tailor interactions based on individual patient needs, preferences, and health history. This can lead to more engaging and meaningful conversations that resonate with patients on a personal level.
Second, multimodal interfaces are expected to gain traction. These interfaces combine voice, text, and visual elements to create a more comprehensive communication tool. By employing various modes of interaction, healthcare providers can offer adaptability to different patient demographics and preferences.
Third, the integration of AI with wearable technology is a noteworthy trend. Devices like smartwatches and fitness trackers can relay real-time health data to conversational AI systems, facilitating proactive health management and timely responses from healthcare professionals.
Moreover, there is a growing emphasis on interoperability among different healthcare systems. Ensuring that conversational AI platforms can seamlessly communicate with electronic health records and other digital health tools will be crucial for gathering holistic patient information.
Prospective Innovations
Looking ahead, several innovations promise to shape the effectiveness of clinical conversational AI. One such innovation is improved Natural Language Processing (NLP) capabilities. Advances in NLP will enable conversational systems to better understand and respond to complex medical queries. This will enhance the systems’ capacity to provide accurate information and recommendations tailored to each patient's situation.
Another promising innovation is the potential for adaptive learning systems. Through machine learning, conversational AI can analyze interaction patterns, identify areas for improvement, and evolve to meet emerging healthcare demands. This will help in continuously refining patient interactions to ensure relevance and efficacy.
Furthermore, the incorporation of emotion recognition technology is on the horizon. This technology aims to recognize and respond to a patient's emotional state during interactions. By analyzing verbal and non-verbal cues, AI systems can provide more empathetic responses, thus strengthening the patient-provider relationship.
"The future of clinical conversational AI hinges on innovation, personalization, and the ability to seamlessly integrate with existing systems."
The End and Recommendations
The conclusion of this article synthesizes the intricate aspects discussed throughout, emphasizing the significance of clinical conversational AI in transforming healthcare communication. It is clear that the advent of conversational AI has profound implications for patient engagement, operational efficiency, and data management within the healthcare sector. These advancements not only enhance the experience for patients but also streamline processes for healthcare providers, ultimately contributing to improved outcomes.
Strategic Implementation Approaches
Implementing clinical conversational AI systems requires a well-thought-out strategy. Here are some key approaches:
- Assessment of Needs: Organizations must first evaluate their specific needs. This includes understanding what problems conversational AI can solve within their current systems, whether improving patient interaction or handling administrative tasks.
- Integration with Current Systems: It’s crucial for conversational AI to work seamlessly with existing healthcare systems. This ensures that there is no disruption in operations and that staff can easily adapt to new technologies.
- Training and Education: Ongoing training for healthcare personnel using conversational AI is essential. This fosters confidence in the technology and improves utilization rates.
- Continuous Monitoring: Post-implementation, it’s vital to monitor AI interactions and outcomes continuously. This helps identify areas for improvement and ensures the system evolves in response to user feedback.
- Compliance and Security: Organizations must ensure that any AI system adheres to healthcare regulations concerning data privacy and security. This is critical, as healthcare data is particularly sensitive.
Future Research Directions
The landscape of clinical conversational AI is ever-evolving, and several future research directions can enhance its application:
- User Experience Studies: More in-depth studies on user experience can refine how these systems interact with patients, ensuring that the communication is not only efficient but also empathetic.
- Broader Dataset Development: Future research should focus on creating larger and more diverse datasets. This will improve the AI’s ability to understand and respond to different patient demographics.
- Interdisciplinary Approaches: Collaboration between AI technologists, healthcare professionals, and policymakers can lead to innovations that respect both technological capabilities and medical ethics.
- Longitudinal Impact Studies: Researching the long-term impact of conversational AI on patient outcomes will provide valuable insights into its effectiveness and inform best practices.
In summary, the conclusion and recommendations section encapsulates the transformative potential of clinical conversational AI while advising on effective implementation strategies and future research initiatives. By addressing these areas, healthcare organizations can harness the power of AI to foster meaningful communication and ultimately improve patient care.