Cutting-Edge Conversational AI Technologies Uncovered

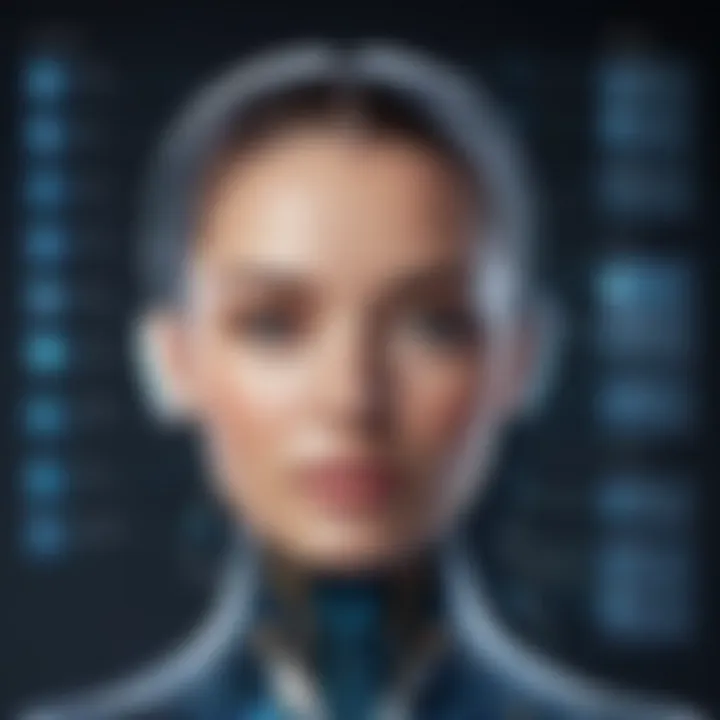
Intro
The field of conversational AI has recently experienced substantial developments, reshaping how businesses and individuals communicate. It is no longer sufficient to rely on basic programmed responses; users expect more nuanced and intuitive interactions. This evolution leads us to explore the advanced conversational AI technologies that define the current landscape.
The primary goal of this article is to delve into the capabilities of existing platforms, examining their structures and the significance they hold across various industries. Additionally, we will analyze innovative trends, ethical dilemmas, and a comparative assessment of leading solutions in the realm of conversational AI. Through this investigation, readers will gain practical insights that empower business decision-makers and tech enthusiasts to navigate a rapidly evolving sector effectively.
Prelims to Conversational AI
Conversational AI refers to technologies that enable machines to engage in human-like dialogue. The development of these technologies has become paramount in today's digital landscape. It affects various sectors, making communication seamless and interactive. From customer service to personal assistants, conversational AI redefines user experience and operational efficiency.
In this article, we will explore the multitude of dimensions in conversational AI. We will uncover its foundational components, historical context, and technological advancements. The insights provided aim to equip both tech enthusiasts and business decision-makers with an understanding of how these systems function and their implications on various fields.
Definition and Scope
Conversational AI encompasses a range of technologies that facilitate communication between humans and machines. This includes voice-based assistants, chatbot systems, and interactive voice response (IVR) systems. The scope is broad, focusing not only on verbal communication but also on written exchanges. Natural Language Processing (NLP) and speech recognition are pivotal in this area.
- Natural Language Processing (NLP): This is the core of conversational AI. It allows machines to understand, interpret, and respond to human language in a way that is both meaningful and contextually relevant.
- Machine Learning: Central to advancing conversational AI, machine learning algorithms help systems to learn from data, improving responses over time based on user interactions.
- Speech Recognition: This technology enables the conversion of spoken language into text, facilitating interaction through voice commands.
The combination of these elements creates a robust platform for conversational interfaces, extending their applicability across various businesses and industries.
Historical Development
The evolution of conversational AI can be traced back several decades. It began with rudimentary chatbots designed to respond to simple queries. Early examples include ELIZA, developed in the 1960s, which simulated a psychotherapist using pattern matching. Over the years, advancements in computer science and linguistics propelled the development further.
The 1990s saw more sophisticated voice recognition software emerging, signaling a shift toward interactive systems. As the new millennium approached, innovations in machine learning and data analysis led to significant improvements in NLP, enhancing the understanding of context and conversation flow.
Fast forward to the present day, and we see powerful platforms such as Google Dialogflow and IBM Watson Assistant leading the frontier of conversational AI. These platforms not only process language but also integrate seamlessly with various applications, making them integral to business strategy today.
As we delve deeper into this article, we will examine key technologies, leading platforms, their architecture, and real-world applications, all of which underscore the growing relevance of conversational AI.
Key Technologies Driving Conversational AI
Conversational AI has transformed how businesses interact with users. It relies on several key technologies that work together to create efficient, natural, and meaningful dialogues. Understanding these technologies is crucial for anyone involved in deploying or managing conversational AI solutions. They offer various benefits, such as improved user engagement and operational efficiency.
Natural Language Processing
Natural Language Processing, or NLP, is the backbone of any conversational AI system. It allows machines to understand, interpret, and respond to human language in a meaningful way. NLP encompasses several techniques, including text analysis, sentiment analysis, and language generation.
One of the main benefits of NLP is its ability to process large amounts of text data efficiently. This capability enables systems to understand user queries and provide relevant responses quickly. It is not just about keyword recognition; it involves understanding context, intent, and even emotion behind the words.
For instance, an AI model trained in NLP can differentiate between statements like "I am sad" and "I am happy," enabling it to respond appropriately. Additionally, NLP supports multiple languages, enhancing accessibility for global users.
Machine Learning Algorithms
Machine Learning algorithms enhance conversational AI by enabling systems to learn from interactions over time. These algorithms analyze user input, recognize patterns, and predict future responses. With ongoing learning, the accuracy of responses continues to improve, making the AI more effective over time.
Various algorithms are used, including supervised and unsupervised learning methods. Supervised learning involves training the AI on labeled data, while unsupervised learning allows it to identify patterns without pre-existing labels. This adaptability is vital in rapidly changing business environments, where user expectations can shift.
Benefits of integrating Machine Learning into conversational AI include:
- Increased user satisfaction through personalized interactions.
- Reduction in required human oversight as the systems self-improve.
Speech Recognition
Speech Recognition technology allows conversational AI systems to comprehend spoken language. This technology not only captures speech but also converts it into text, which can be processed further by NLP modules.
Accuracy in speech recognition relies heavily on the system's ability to detect accents, dialects, and nuances in pronunciation. Voice commands have become integral in various applications, from virtual assistants like Google Assistant to smart speakers. By using advanced algorithms, systems can now understand contextual phrases and reduce errors significantly compared to older methods.
The key advantages of speech recognition technology include:
- Hands-free interaction, which enhances user experience while multitasking.
- Increased accessibility for those with disabilities or those preferring voice over text inputs.
Leading Conversational AI Platforms
Leading conversational AI platforms play a pivotal role in the development and deployment of intelligent systems that enhance communication between humans and machines. These platforms offer tools and frameworks that allow designers and developers to build robust conversational agents. They facilitate dynamic interactions and improve usersβ experience across various digital touchpoints.
Understanding these leading platforms is crucial for businesses aiming to implement conversational AI. They provide essential capabilities like natural language understanding, context management, and integration with existing systems, making it easier to adopt AI technologies. Whether for customer service, personal assistance, or operational automation, the potential impact of these platforms in transforming business processes cannot be understated.
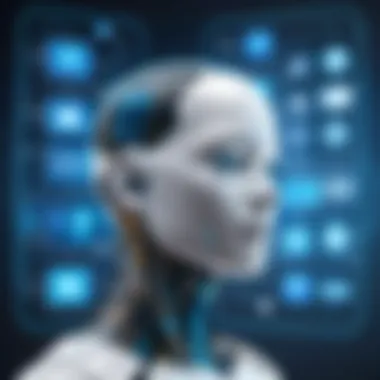
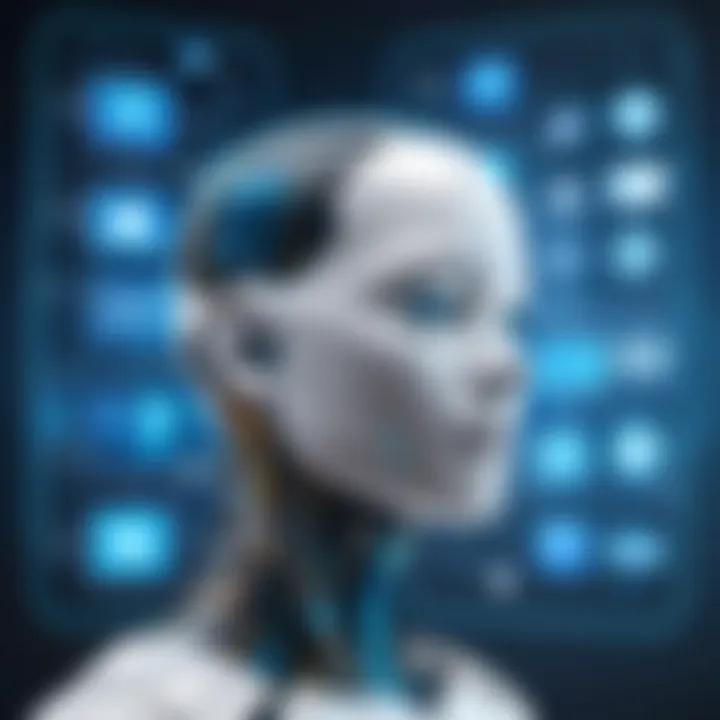
Microsoft Azure Bot Services
Microsoft Azure Bot Services enables developers to create, test, and deploy intelligent bots. It offers a comprehensive framework built on top of Azure cloud services, integrating with various AI capabilities like natural language processing and machine learning. The platform supports multiple channels, including Microsoft Teams, Slack, and Facebook Messenger, allowing seamless deployment across various platforms.
In terms of functionality, it allows for customization through the Bot Framework SDK, giving developers the tools they need to create specific solutions. Security and compliance are built into Azure services, ensuring user data is handled responsibly. Moreover, the analytics capabilities provide insights into user interactions, enabling continuous improvement.
Google Dialogflow
Google Dialogflow is a natural language understanding platform that empowers applications with the ability to engage in conversations with users. Its capabilities extend from simple text-based interactions to voice recognition. The platform excels in processing intents and entities, which allows for a more nuanced understanding of user queries.
Dialogflow's integration with Google Cloud enhances its functionalities. It leverages Googleβs extensive machine learning infrastructure to continuously improve its algorithms based on user input. Its easy-to-use interface makes it accessible for developers at various skill levels, making it a popular choice in different industries aiming to enhance their customer interaction strategies.
IBM Watson Assistant
IBM Watson Assistant stands out for its focus on enterprise solutions. The platform enables businesses to build AI-driven agents that can assist customers across various channels. IBM's robust natural language processing capabilities allow for advanced understanding of user inquiries, enabling more accurate responses.
Moreover, Watson Assistant can integrate with business systems, providing context-driven support that fits into the workflow of organizations. It features tools for tracking user interactions and insights that assist in refining the conversational experience. This capability allows businesses to leverage AI for meaningful and insightful customer service.
Amazon Lex
Amazon Lex, part of the AWS ecosystem, provides an advanced engine for building conversational interfaces with voice and text. It integrates seamlessly with other AWS services, facilitating easy deployment of chatbots and virtual assistants. Lexβs strengths lie in its conversational design and the ability to integrate speech recognition.
Amazon Lex is used in various applications, from simple FAQ bots to complex systems that require nuanced user interaction. The ability to build sophisticated models with minimal resources makes it accessible for startups and established companies alike. Continuous updates and improvements are a hallmark of Amazon's commitment to providing cutting-edge solutions.
"The landscape of conversational AI is constantly evolving, and leveraging these platforms is key for any organization aiming to stay competitive in today's market."
In summary, leading conversational AI platforms like Microsoft Azure Bot Services, Google Dialogflow, IBM Watson Assistant, and Amazon Lex each offer unique strengths that can cater to different business needs. By understanding these platforms, businesses can make informed decisions on which solution best aligns with their goals and technological infrastructure.
Architecture of Conversational AI Systems
The architecture of conversational AI systems serves as the foundation that enables these technologies to function effectively. Understanding this architecture is essential for businesses hoping to implement conversational AI solutions. It encompasses not only the technological components but also the design principles that make these systems user-friendly and efficient.
A well-designed architecture provides scalability, interoperability, and the ability to integrate with other systems. These aspects are particularly significant as organizations seek to customize solutions that fit their specific needs and workflows. Additionally, a clear architecture helps in debugging and upgrading the system, ensuring longevity and adaptability in a fast-changing technology environment.
Understanding the Components
Conversational AI systems consist of several interlinked components that work together to generate meaningful interactions. The primary components include:
- Natural Language Processing (NLP): This enables the system to comprehend and interpret human language, making it possible to process queries accurately.
- Dialogue Management: This component manages the flow of conversation and determines the next steps in the dialogue based on user input.
- Speech Recognition: This allows the system to convert spoken language into text, facilitating real-time interactions.
- User Interface: This includes all touchpoints where the user interacts with the AI, which can range from chatbots to voice assistants.
- Data Storage: Storing user data, historical conversations, and training datasets is critical for improving responses and personalizing the user experience.
Each of these components plays a vital role in ensuring that the conversational AI system delivers accurate and relevant information while maintaining user engagement. For instance, advancements in NLP enhance a systemβs ability to understand context and sentiment, leading to more natural interactions.
User Interface Design Considerations
The user interface (UI) design is a critical aspect of conversational AI systems, as it greatly influences user experience. A well-thought-out UI ensures that users can interact with the AI effectively. Key considerations for UI design include:
- Simplicity: A straightforward design minimizes user frustration and enhances accessibility.
- Feedback Mechanisms: Integrating mechanisms for users to provide feedback helps improve the system continuously.
- Contextual Awareness: A UI that adapts based on the userβs input context leads to a more personalized experience.
- Multimodal Interaction: Supporting voice, text, and visual elements can cater to user preferences and contexts.
- Consistency: Ensuring a consistent design across various platforms helps in building familiarity and ease of use.
An effective UI can significantly reduce user resistance to interacting with AI systems. Thoughtful design can encourage adoption and enhance the perceived value of conversational AI technologies.
"The architecture and user interface of conversational AI systems are crucial for maximizing user engagement and achieving business objectives."
Applications and Use Cases
Understanding the diverse applications and use cases of conversational AI technologies is crucial for grasping their impact on modern businesses and consumer interactions. These technologies have become advantageous tools across different industries. They revolutionize customer engagement and streamline operations. By automating responses and facilitating communication, organizations can enhance their effectiveness and improve user experiences. In this section, we will examine three prominent application areas: customer support automation, e-commerce solutions, and healthcare assistants.
Customer Support Automation
Customer support has transformed with the advent of conversational AI. Businesses can utilize chatbots and virtual assistants to handle inquiries efficiently. These systems offer immediate responses, reducing wait times for customers significantly. Additionally, they can manage high volumes of interactions without diminishing service quality.
Conversational AI in customer support can also lead to cost savings. Human agents can focus on more complex issues while AI handles routine questions. This is particularly useful in environments with repetitive queries, such as FAQs or order tracking. The increased efficiency ensures that responses are accurate and timely.
"Automation in customer support helps enhance user satisfaction and optimizes resource allocation."
Key benefits include:
- 24/7 Availability: Customers receive support at any time, improving overall satisfaction.
- Scalability: AI can handle varying amounts of customer inquiries without additional staff.
- Data Collection: These systems gather valuable insights about customer behavior, enabling targeted solutions.
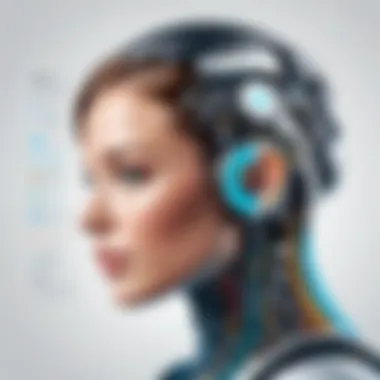
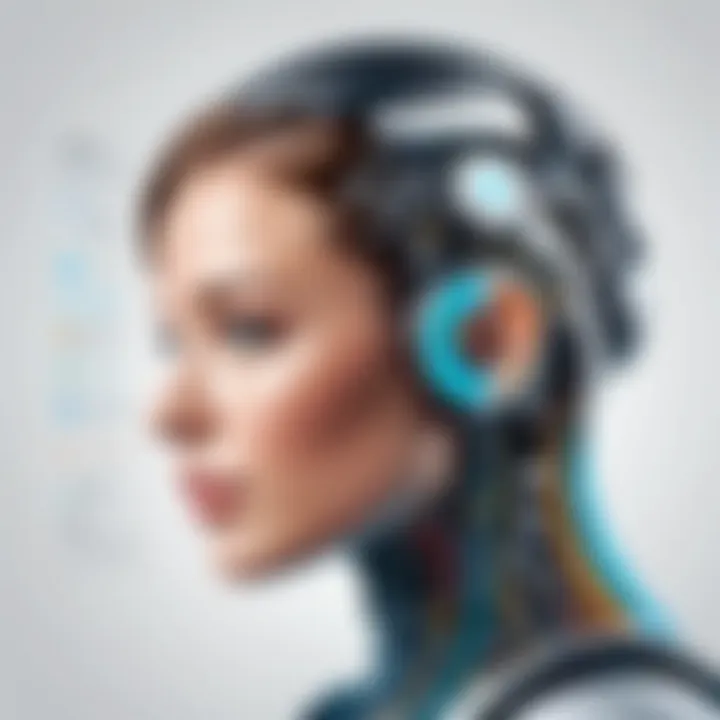
E-commerce Solutions
E-commerce has seen substantial innovation through conversational AI. Online retailers utilize chatbots to guide customers through the purchasing journey. Whether it is personalized product recommendations or assisting with checkout, these AI-driven tools create a smoother shopping experience.
Moreover, by implementing conversational AI, businesses can enhance upselling and cross-selling. Chatbots can suggest additional items based on a customer's preferences. This tailored approach not only increases sales but also nurtures customer loyalty.
Key aspects include:
- Personalization: AI enhances user experiences through customized interactions.
- Order Management: Buyers can easily track their orders and initiate returns.
- Improved Engagement: Conversational AI fosters interactive communication that leads to higher engagement rates.
Healthcare Assistants
In healthcare, conversational AI is invaluable. Virtual health assistants help streamline processes for patients and healthcare providers. From booking appointments to providing medication reminders, AI improves operational efficiency. Patients can access information quickly, which is critical in urgent situations.
Additionally, conversational AI facilitates telemedicine. Patients can consult with healthcare professionals via chatbots that triage symptoms and provide general health information. This accessibility can help reduce the burden on healthcare services.
Important points include:
- Accessibility: Patients can access health information at any time, enhancing patient engagement.
- Cost-Effectiveness: Reduces operational costs associated with patient inquiries.
- Data-Driven Insights: AI systems can analyze patient interactions and improve service offerings.
Impact on Business Operations
Conversational AI technologies play a significant role in reshaping business operations. As organizations strive for efficiency and responsiveness, integrating these systems into their workflows can provide substantial advantages. This section focuses on how conversational AI influences various aspects of business, particularly through efficiency improvements and cost reduction strategies.
Efficiency Improvements
One of the most prominent benefits of conversational AI is the enhancement of operational efficiency. Automating routine tasks allows businesses to redirect human resources toward more strategic activities. Conversational AI systems can handle numerous inquiries concurrently, reducing wait times for customers and improving response rates.
For instance, chatbots can manage customer interactions outside of typical business hours, ensuring service continuity. This availability leads to enhanced customer satisfaction, fostering loyalty and repeat business. Efficient systems also allow organizations to gather and analyze data swiftly, which supports informed decision-making.
The integration of AI into customer service platforms has shown remarkable results. By employing solutions like Microsoft Azure Bot Services or IBM Watson Assistant, many companies have streamlined their operations. These tools utilize intelligent automation to handle inquiries, direct customers to the right resources, and even escalate complex issues to human operators as necessary.
As a direct result of efficiency gains from conversational AI, businesses can provide better quality service while optimizing various internal processes. Employees can focus on creative problem-solving and innovation instead of routine questions or issues that AI can address.
Cost Reduction Strategies
Cost efficiency is another significant factor that drive organizations to adopt conversational AI technologies. The initial investment in these systems can be mitigated through various operational savings. Reducing labor costs is often the primary focus; businesses can serve more customers with fewer staff. This model allows for a more flexible allocation of human resources where they are most needed.
Additionally, companies can minimize costs related to customer support and operational overhead. For example, by using Google Dialogflow, organizations can develop tailored support solutions without the extensive expenses of building out entire call centers. Strategies that integrate conversational AI often lead to savings on software and support resources as well.
"Conversational AI can help streamline support processes, leading to significant cost savings for businesses within a short time frame."
Moreover, maintaining efficiency through these tools can lead to lower churn rates, which directly affects revenue. Improved customer experience means less spending on retention strategies and re-engagement campaigns. Overall, the smart deployment of conversational AI results in a compelling business case centered around cost reduction.
In summary, the impact of conversational AI on business operations cannot be understated. The balance between efficiency improvements and cost reduction strategies creates a compelling reason for businesses to invest in these technologies.
Ethical Considerations in Conversational AI
Given the rapid evolution of technology, it comes as no surprise that ethical considerations surrounding conversational AI have emerged as a significant area of concern. These technologies influence how users interact with systems and each other in various contexts. Understanding the ethical implications is crucial, not only for better designs but also for maintaining user trust and compliance with regulations. This section addresses two primary components: data privacy issues and bias in AI training data, shedding light on the potential pitfalls and stresses the necessity of addressing them.
Data Privacy Issues
Data privacy is at the forefront of ethical discussions in AI. Conversational AI systems often process large amounts of user data to provide personalized experiences. However, this data can include sensitive information that, if mishandled, poses significant risks to individuals and organizations.
When users interact with AI, they may unknowingly share personal information. Providers must implement strict guidelines on data handling. Proper consent must be obtained before data collection. This creates an environment of trust, encouraging users to engage honestly with these systems.
Moreover, ensuring compliance with data protection regulations such as GDPR in Europe and CCPA in California is essential. Non-compliance can lead to severe penalties. Therefore, organizations utilizing conversational AI must prioritize data security measures. This includes implementing robust encryption, limiting data access, and regularly auditing systems to identify vulnerabilities.
"Protecting user data is not just a regulatory obligation; itβs a fundamental aspect of ethical AI interaction."
Implications of Poor Data Privacy Management
- Loss of user trust
- Legal repercussions and fines
- Damage to brand reputation
Overall, businesses must develop transparent data handling procedures. Educating users about how their data will be used is a vital step in creating an ethical framework for conversational AI.
Bias in AI Training Data
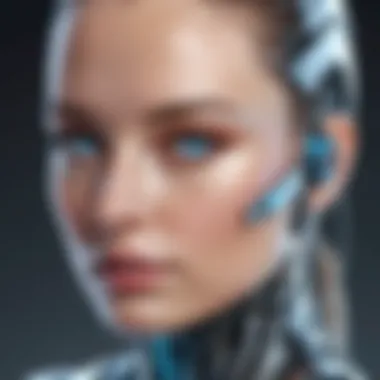
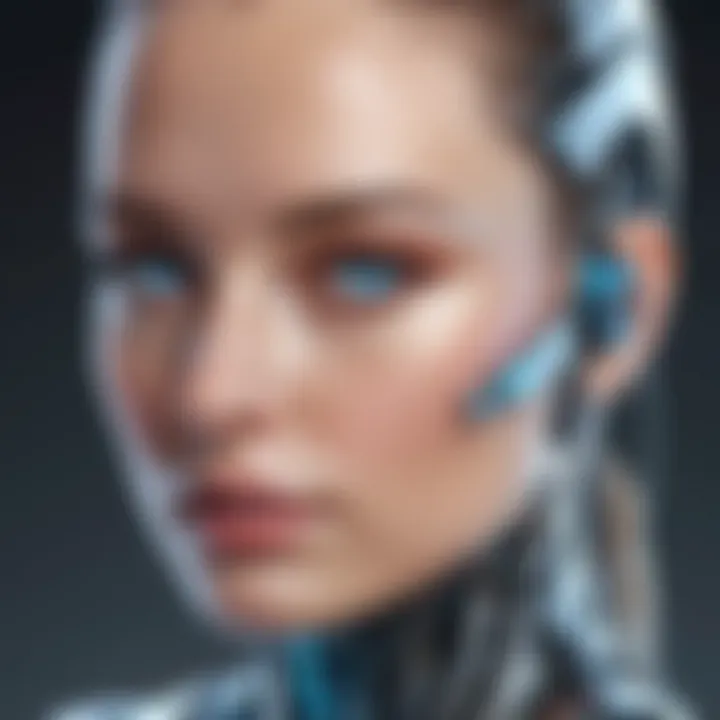
Bias in AI training data represents another critical ethical challenge. AI systems learn from historical data, which may contain inherent biases. If these biases are not addressed, they can perpetuate discrimination in outcomes. This has significant implications for diverse fields such as hiring, lending, and law enforcement.
Addressing bias requires thorough audits of datasets used for training. It is essential to ensure that the data is representative of the broader population. Failing to do so can lead to skewed results and decisions made by the AI that reflect past inequalities rather than reality.
Additionally, organizations need to implement continuous evaluation techniques. This involves regularly testing AI models for biases and adjusting algorithms when necessary. Engaging a diverse group of developers and stakeholders can also provide valuable insights into potential blind spots in AI behavior.
Key Strategies to Mitigate Bias
- Regularly reviewing and refining training datasets
- Implementing fairness-enhancing technologies
- Engaging diverse teams in the development process
Challenges and Limitations
The exploration of conversational AI technologies is not devoid of challenges and limitations. This aspect is crucial in understanding the full scope of what these systems can achieve and the potential setbacks that may arise. Addressing these challenges promotes a more effective implementation of AI technologies while ensuring they meet user and organizational needs adequately.
Understanding Contextual Nuances
One significant challenge relates to the understanding of contextual nuances in human conversation. Conversational AI systems often struggle with context, which can lead to misunderstandings and errors in response. In natural language, context can shift rapidly based on previous interactions, sarcasm, or cultural references. If a system fails to grasp these subtleties, it risks providing irrelevant or incorrect information should also be capable of recognizing when the conversation's context has changed.
This limitation poses various implications for user experience. Users expect conversational agents to be context-aware, responding appropriately to previous exchanges. Thus, an AI lacking this capability may frustrate users, leading to decreased engagement and trust in the system. Furthermore, as these technologies are integrated into customer service or healthcare applications, the stakes become higher. Misinterpretations could result in poor service or even risks to health. Therefore, developers must focus on enhancing contextual understanding within AI systems. This involves employing advanced natural language processing techniques and machine learning algorithms capable of learning from interactions over time.
Maintaining User Trust
Maintaining user trust is another pressing challenge in conversational AI. Trust is foundational in any user-system interaction; if users do not trust the AI, they will not engage with it properly. Trust can be compromised in various ways, primarily through privacy concerns and a lack of transparency.
For instance, users often worry about how their data is being utilized. Conversational AI collects vast amounts of personal information, and any misstep in data handling can lead to privacy violations. Transparency regarding data use policies and implementing robust security measures can go a long way in building trust.
Moreover, the interpretation of responses and the reliability of information provided by AI systems also play a critical role. If users perceive that the AI system provides inconsistent or erroneous answers, they are likely to lose faith in its capabilities. It, therefore, becomes the duty of developers and organizations to create solutions that are not only accurate but also provide explanations for their responses whenever possible. This approach can foster a sense of reliability among users.
In summary, addressing the challenges of understanding contextual nuances and maintaining user trust is essential for the future of conversational AI technologies. By focusing on these limitations, developers can enhance user experience and leverage the full potential of conversational AI.
Future Trends in Conversational AI
The future of conversational AI is increasingly becoming a focal point for businesses and technology enthusiasts alike. As the landscape evolves, several trends are emerging that are not only reshaping user interactions but also influencing strategic decisions across industries. Understanding these trends is critical, as they serve to highlight the potential benefits, challenges, and the necessary considerations organizations must navigate in adopting these advanced technologies.
Advancements in Emotional AI
Advancements in emotional AI signify a leap toward creating more nuanced and empathetic interactions. Emotional AI aims to enable machines to recognize and respond to human emotions through various means, such as natural language processing and sentiment analysis. This capability is essential in customer service applications, where understanding customer feelings can enhance satisfaction and promote loyalty.
Key benefits of integrating emotional AI include:
- Enhanced User Experience: A more personalized interaction can lead to better user engagement and satisfaction. For instance, chatbots that recognize frustration may escalate issues to human agents faster.
- Improved Customer Engagement: By tailoring responses based on emotional cues, businesses can create more meaningful connections with users.
- Data-Driven Insights: Organizations gain insights about customer sentiment which can inform service improvements and marketing strategies.
Incorporating emotional intelligence in conversational systems, however, is not without its challenges. Developers must be cautious of privacy concerns and the ethical implications of interpreting and responding to human emotions.
Integration with IoT Devices
The integration of conversational AI with Internet of Things (IoT) devices represents another significant trend. This synergy enables a seamless interaction between users and their connected devices, enhancing functionality and user experience. For example, smart home assistants, like Amazon Alexa or Google Nest, use conversational AI to control lighting, temperature, and security systems through simple voice commands.
Benefits of this integration include:
- Efficiency: Users can streamline daily tasks, offering increased convenience.
- Interoperability: Devices can communicate effortlessly, creating a more cohesive smart home ecosystem.
- Real-time Responses: Immediate feedback and control enhance users' efficiency in managing multiple devices.
Despite these advantages, there are considerations to keep in mind. The complexity of integrating diverse technologies might pose technical challenges. Furthermore, addressing security concerns is paramount, as an increased number of connected devices could expose systems to potential threats.
The convergence of emotional AI and IoT opens doors to unprecedented levels of automation and user satisfaction, calling for a thoughtful approach to design and implementation.
Ending
The discussion surrounding conversational AI technologies is vital for understanding their profound implications in various sectors. As we draw this article to a close, it is essential to synthesize the insights gathered throughout. Conversational AI is not merely a trend; it represents a shift in how we interact with technology and each other. The advent of sophisticated platforms and algorithms has paved the way for enhanced user experiences and increased operational efficiencies.
Summary of Insights
During this exploration, we have delved into several key elements:
- Technological Foundations: The underlying technologies, such as natural language processing and machine learning, are critical for the functionality of conversational AI. Their evolution has made systems more efficient and capable of complex interactions.
- Impact on Industries: Various sectors including healthcare, e-commerce, and customer support have seen significant benefits from the integration of conversational AI. These systems streamline operations, reduce costs, and enhance user satisfaction.
- Ethical Challenges: The ethical considerations surrounding data privacy and biases in AI training have emerged as pressing issues. Organizations must navigate these challenges carefully to foster trust with users.
- Future Outlook: We have also discussed future trends, like the significance of emotional AI and the integration with IoT devices, which suggest continuing advancements in this technology.
"Exploring the nuances of conversational AI can not only enhance our understanding but also highlight areas needing attention for future advancements."
Final Thoughts on Adoption
Adopting conversational AI requires careful consideration and strategy. Organizations should assess their specific needs and align the chosen technology accordingly. Key points for successful adoption include:
- Customization: Tailoring solutions to fit unique business models ensures better results.
- User Experience: Prioritizing an intuitive user interface is crucial for adoption and long-term success.
- Continuous Monitoring: Implementing a feedback loop can inform further improvements and adjustments post-adoption.
In summary, conversational AI has the potential to redefine various industry landscapes, yet it demands a thoughtful approach to effectively harness its advantages. Decision-makers and IT professionals must stay abreast of trends and ethical concerns to facilitate responsible and innovative adoption.