Unlocking Business Potential with RL Solutions
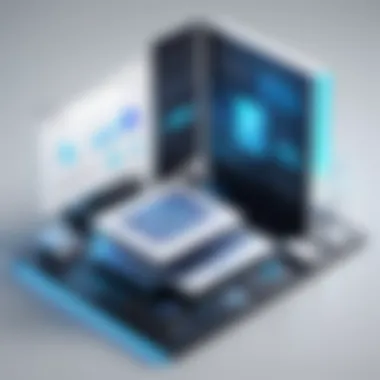
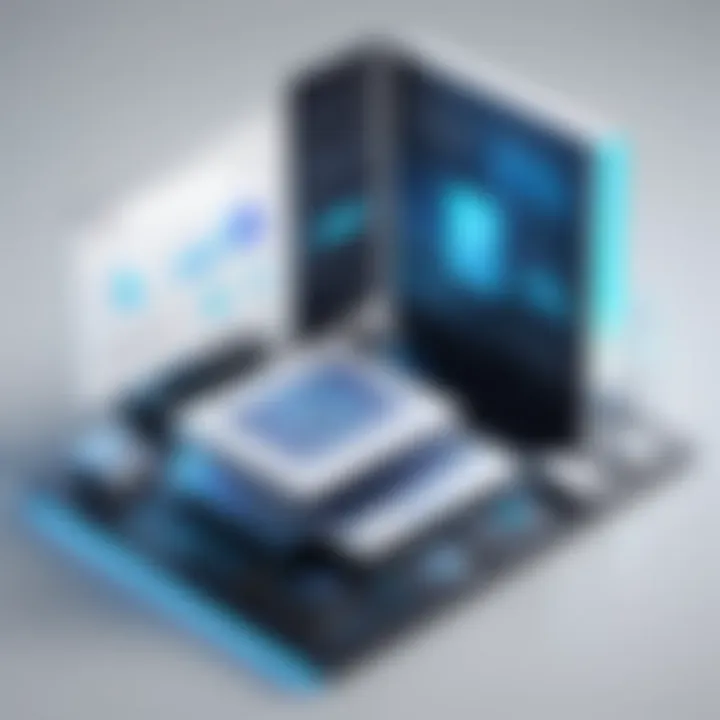
Intro
In the evolving landscape of B2B software, Reinforcement Learning (RL) solutions have emerged as a pivotal element driving operational efficiency and strategic decisions. The growing acknowledgment of data-driven methodologies among businesses signals a shift towards more intelligent applications. This article examines the intricacies of RL solutions, delving into their roles, advantages, and practical implementations tailored specifically for small to medium-sized enterprises (SMEs).
Software Overview
Software Description
Reinforcement Learning refers to a category of artificial intelligence that enables software systems to learn optimal actions through trial and error. In a bustling B2B environment, RL solutions empower businesses to refine their processes, reduce operational costs, and enhance customer satisfaction. These systems frequently integrate with existing software applications, enabling data analysis and decision-making at enhanced levels.
Key Features
The capabilities of RL solutions resonate in various domains such as supply chain management, customer relationship management, and sales optimization. Here are the key features that set them apart:
- Adaptive Learning: RL systems adjust to new data automatically, allowing for continuous improvements in performance over time.
- Predictive Analytics: These solutions utilize historical data to predict future outcomes, which is crucial for strategic planning.
- Dynamic Decision-Making: The ability to make real-time decisions can drastically improve responsiveness to market changes.
- Personalization: RL can be used to tailor experiences and solutions to individual customer needs, boosting client engagement.
"Incorporating RL into B2B software can lead to greater innovation and a competitive edge."
Pricing Models
Subscription Options
Many vendors opt for subscription-based pricing. This model allows businesses to access software without a hefty initial investment. Subscriptions typically come with several tiers, meaning businesses can select a plan that aligns with their operational scale. Features include:
- Regular updates and support services.
- Flexibility to scale usage based on growing business needs.
- Reduced financial risk as costs can be spread over time.
One-Time Purchase
Alternatively, some solutions are available under a one-time purchase model. This may appeal to companies looking for long-term stability without ongoing costs. However, businesses must be prepared for:
- Higher upfront costs.
- Additional expenses for upgrades and support, which may arise from lack of recurring payments.
- Potential challenges adapting to new technology or training employees without vendor assistance.
Each pricing model carries its advantages and disadvantages. Decision-makers must assess their unique circumstances and requirements in determining the most suitable approach.
As we analyze RL solutions within the B2B software framework, it becomes evident how these tools facilitate significant advancements in operational efficiency. The evolving complexities of business demand tailored solutions that not only meet immediate needs but also adapt over time, firmly positioning RL as an indispensable player in this domain.
Intro to RL Solutions
The discussion surrounding Reinforcement Learning (RL) solutions is more relevant than ever in the domain of business-to-business (B2B) software. As companies seek to enhance operational efficiencies and automate strategic processes, the role of RL in achieving these goals cannot be understated. RL solutions draw on complex data-driven algorithms to refine their performance over time, proving invaluable for decision-makers pondering over their integration into enterprise workflows.
In this section, we will present the definitions and historical context of RL solutions. Understanding these foundational aspects will provide clarity on why this technology has become a focal point for B2B software developers and businesses alike.
Definition of RL Solutions
Reinforcement Learning solutions represent a subset of machine learning techniques that focus on how software agents ought to take actions in an environment to maximize cumulative rewards. Rather than being provided with explicit instructions, these agents learn through trial and error, adapting their strategies based on feedback from their actions.
This unique approach is what differentiates RL from other machine learning paradigms. It allows for dynamic decision-making, immensely useful in scenarios where outcomes are uncertain or depend on the actions of other agents within the system. With the capability to process vast amounts of data and learn from it effectively, RL solutions can significantly increase the efficiency of B2B operations.
Historical Context
The roots of Reinforcement Learning can be traced back to the early days of AI research in the 1950s and 1960s. Pioneers like Richard Sutton and Andrew Barto laid the groundwork, exploring concepts that would evolve into RL frameworks. Fast forward to the 21st century, technological advancements and increased computational power burst open the doors for RL applications in various fields.
Notable milestones include the introduction of algorithms such as Q-learning and Deep Q-Networks, which enabled RL models to tackle more complex environments. The successes of RL in games like Go, where AI defeated human champions, signified a major turning point, catching the attention of industries looking for innovative solutions.
Today, RL solutions are not merely theoretical concepts but practical tools that have proven their worth across sectors. They harness the strengths of artificial intelligence to address real B2B challenges, shaping the future of software capabilities in significant ways.
Importance of RL Solutions in B2B
Reinforcement Learning (RL) solutions are becoming increasingly crucial in the B2B software landscape. Companies are now embracing machine learning and artificial intelligence to optimize their operations and enhance decision-making capabilities. The integration of RL into business processes offers distinct advantages that cater to the unique challenges faced by organizations today. These benefits are particularly relevant for small to medium-sized enterprises who seek efficiency and competitive edge.
Strategic Advantages
One of the primary reasons businesses adopt RL solutions is the numerous strategic advantages they offer. RL algorithms simulate learning processes that allow systems to improve over time based on feedback received from their environment. Here are some key advantages:
- Operational Efficiency: RL solutions streamline processes and reduce operational costs. By automating complex decision-making tasks, businesses can focus on core competencies.
- Personalization: These solutions can tailor services and products to individual customer preferences, resulting in improved customer satisfaction and loyalty.
- Predictive Capabilities: With their ability to analyze large datasets, RL solutions can offer predictive insights that guide strategic planning. This helps businesses to adapt quickly to ever-changing market conditions.
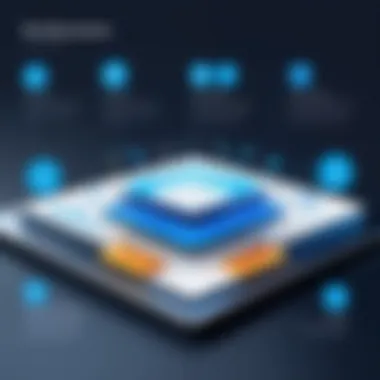
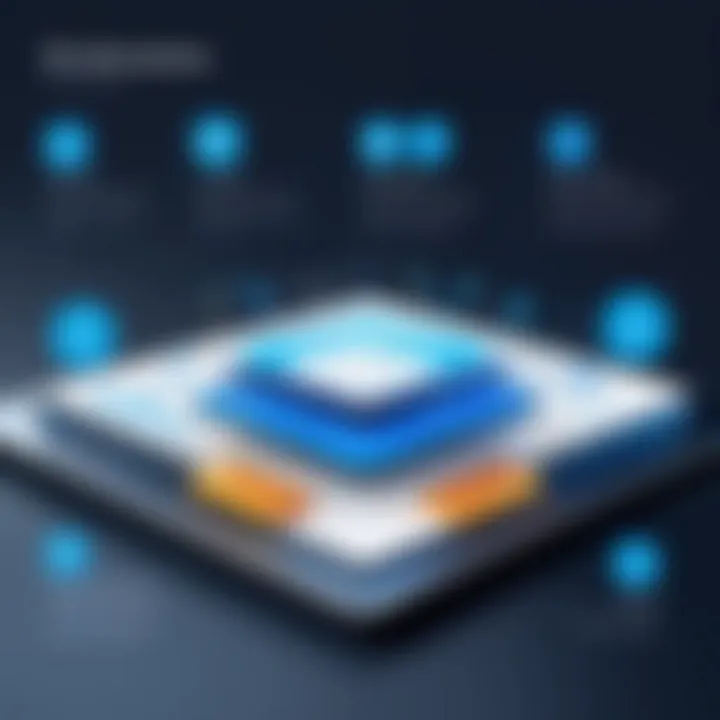
Implementing RL not only aids in current operations but also positions a company for future growth. This adaptability is vital in the fast-paced business environment.
Impact on Decision-Making
The impact of RL on decision-making cannot be understated. Effective decision-making requires accurate data analysis, forecasting, and strategy assessment. RL solutions enhance these processes through:
- Data-Driven Insights: RL systems process extensive amounts of data to provide actionable insights. This leads to more informed decision-making across various departments.
- Dynamic Learning: Unlike static models, RL adapts based on new information. It offers organizations the ability to refine decisions in real-time, fostering agility.
- Risk Management: By evaluating potential outcomes of various decisions, RL can help organizations anticipate risks and devise mitigation strategies. This proactive approach minimizes the consequences of poor decisions.
"Organizations leveraging RL solutions can fundamentally elevate their decision-making framework, ensuring more strategic, informed, and timely choices."
Overall, the strategic benefits and enhanced decision-making capabilities brought by RL solutions are instrumental for B2B companies aiming for operational excellence. As these technologies advance, understanding their importance becomes vital for anyone involved in facilitating business growth.
Functionality of RL Solutions
Understanding the functionality of RL solutions is essential for any individual or organization looking to leverage these technologies in the B2B software landscape. These systems provide critical capabilities that enhance operational efficiency and decision-making processes. By defining specific functions and features, businesses can align solutions with their unique operational challenges. This section will explore both core features and the integration of RL solutions with existing systems to provide a comprehensive overview of their functionality.
Core Features
The core features of RL solutions are vital in delivering value to organizations. Some prominent features include:
- Automated Decision-Making: RL solutions use algorithms to make predictions and decisions based on data patterns, reducing the need for manual intervention.
- Adaptive Learning: These systems continuously improve their performance by learning from new data, making them increasingly effective over time.
- Real-Time Analytics: Providing insights into ongoing operations helps organizations respond rapidly to changes and challenges.
- User-Friendly Interfaces: A focus on usability ensures that decision-makers can easily engage with the software, navigating complex data effortlessly.
Incorporating these elements invites efficiency and drives a more effective use of resources. When businesses understand these core functionalities, they are better positioned to select solutions that closely align with their strategic objectives.
Integration with Existing Systems
Integration is a crucial aspect when implementing RL solutions. Many organizations already have established systems in place, and the ability to incorporate new technologies without disruptive changes is highly valued.
Effective integration should meet the following criteria:
- Compatibility: The new RL solution must run on existing software frameworks without extensive modification, ensuring a seamless transition.
- Data Synchronization: It is essential that data from existing systems accurately feeds into the RL solution, creating a cohesive informational ecosystem. This guarantees that decisions are based on comprehensive datasets.
- Scalability: As business needs evolve, the RL solution should scale accordingly. This ensures that future advancements or changes in strategy can be accommodated without starting from scratch.
- Support and Training: Vendors should offer robust support and training to facilitate a smooth integration process, minimizing disruptions and maximizing user adoption.
Evaluating RL Solutions
Evaluating RL solutions is a critical step for any business looking to integrate these systems into their operations. The effectiveness of decision-making processes hinges upon selecting the right tools that align with the unique needs of the organization. Understanding how to assess these solutions can mean the difference between successful implementation and wasted resources.
Careful evaluation aids in identifying the strengths and weaknesses of various options, ensuring that businesses can make informed choices. It is about more than just the initial purchase; it encompasses long-term viability and adaptability to changing market conditions. Thus, proper evaluation fosters not only efficiency but also strategic growth.
Criteria for Selection
When selecting RL solutions, several criteria must be considered to ensure the right fit for your organization. Here are key factors to evaluate:
- Functionality: Does the solution meet specific business needs? Identify the core features that are essential for your operations.
- Scalability: Will the solution grow with your business? Consider whether it can adapt to increasing data and user volumes.
- Integration: Can it integrate smoothly with existing systems? Evaluate compatibility with your current software architecture.
- Vendor Support: What kind of support is offered? Assess the responsiveness and reliability of the vendor's customer service.
- User Experience: Is the interface user-friendly? Gather feedback from potential users to understand their preferences and challenges with the tools.
Incorporating these criteria into the selection process helps clarify expectations and reduces the risk of poor investment decisions.
Cost-Benefit Analysis
A thorough cost-benefit analysis is indispensable when evaluating RL solutions. This process involves not just the monetary costs but also the potential benefits that these systems can provide. Conducting this analysis requires a systematic approach:
- Identify Costs: List all costs involved in purchasing and implementing the RL solution, including licensing fees, maintenance, and training.
- Estimate Benefits: Quantify the benefits that the solution is expected to bring, such as increased operational efficiency, reduced time for decision-making, and enhanced customer satisfaction.
- Consider Intangible Factors: Some benefits may not be quantifiable, such as improved employee morale or better alignment of business processes. These should be noted, as they can significantly impact overall effectiveness.
- Perform a Break-Even Analysis: Calculate how long it will take to realize the benefits relative to the costs. This provides clarity on whether the investment is justified.
- Review Regularly: Technology and market conditions change. Therefore, regularly reviewing the cost-benefit analysis ensures ongoing suitability of the solution.
Conducting a cost-benefit analysis is not merely about crunching numbers; it is about understanding the long-term strategic implications of the investment.
Ultimately, proper evaluation through defined criteria and careful cost-benefit analysis enables organizations to make robust decisions in adopting RL solutions. Doing so paves the way for smoother implementation and greater overall satisfaction with the selected technologies.
Comparative Analysis of RL Solutions
Understanding the comparative analysis of RL solutions is crucial for businesses looking to enhance their operations through technology. Comparing different options allows decision-makers to identify which solution aligns best with their specific needs and objectives. This section aims to dissect the leading software solutions, offering insights that can guide organizations in making informed choices based on their requirements. Considerations include functionality, ease of integration, cost, and support services.
Leading Software Options
When evaluating RL solutions, focusing on the leading software options provides clarity on market offerings. Each vendor possesses unique characteristics and features that may suit different business models, making it paramount to assess these solutions thoroughly.
Vendor A Analysis
Vendor A, known for its advanced algorithmic capabilities, plays a significant role in the market. One of the key characteristics that make Vendor A a favorable choice is its flexibility in deployment. Organizations can easily adapt the software to meet specific use-case requirements. A unique feature of Vendor A is its ability to integrate seamlessly with various existing systems, providing a smooth user experience.

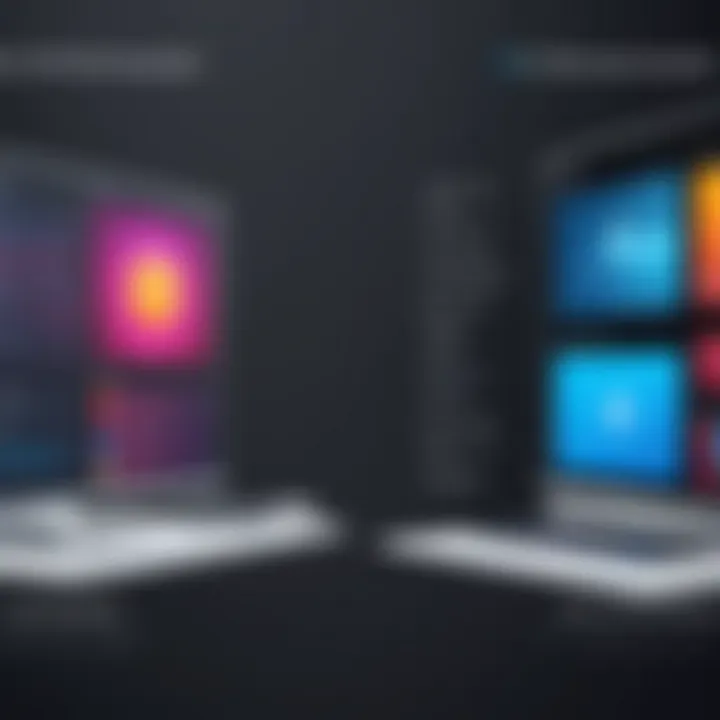
However, some users have pointed out limitations in the customer support service, which can be a drawback when response times are critical. Overall, Vendor A remains a solid option due to its comprehensive functionalities and adaptability to various business environments.
Vendor B Analysis
Vendor B distinguishes itself with strong analytical frameworks that provide businesses with actionable insights. A notable characteristic of Vendor B is its user-friendly interface, which simplifies the navigation processes for all team members. This benefit leads to improved adoption rates within organizations. The unique feature of Vendor B lies in its robust real-time data processing capabilities, allowing organizations to make decisions based on the latest information.
Nonetheless, the pricing structure might not be suitable for smaller enterprises, as costs can escalate quickly depending on the features utilized. Despite this, Vendor B is still popular for businesses prioritizing data analysis and user experience.
Vendor Analysis
Vendor C is recognized for its focus on scalability. Its key characteristic is the capacity to handle large datasets efficiently, making it an option for rapidly growing companies. The software excels in providing extensive customization options, allowing businesses to tailor the solution to fit their evolving needs. A unique feature is its built-in reporting tools, which enhance decision-making processes by keeping stakeholders updated.
On the downside, users may experience a steep learning curve when first adopting Vendor C. The complexity of features could lead to initial integration challenges. However, for organizations planning for long-term growth, Vendor C offers compelling advantages.
Feature Set Comparisons
Comparing the feature sets of leading RL solutions helps organizations visualize where each vendor excels. Important aspects to consider include:
- Integration Capabilities: How easily does the software integrate with existing infrastructure?
- User Interface: Is the software intuitive and accessible for different skill levels?
- Scalability: Can the solution grow with the organization?
- Support Services: What kind of customer support is available post-implementation?
In summary, a thorough comparative analysis of RL solutions can illuminate the best choices for various business types. This process allows decision-makers to leverage software that aligns with their strategic goals.
Case Studies of RL Solutions
Case studies provide real-world examples that illustrate the benefits and challenges of implementing reinforcement learning (RL) solutions in a B2B context. They offer insights that can guide organizations seeking to improve their software applications through the use of RL. Understanding these case studies is essential for decision-makers and IT professionals who aim to enhance operational efficiency and achieve strategic objectives in their enterprises.
Successful Implementations
Several organizations have successfully integrated RL solutions into their business processes, leading to significant improvements. For instance, a logistics company utilized RL algorithms to optimize its supply chain management. By analyzing vast amounts of data, the system made real-time decisions regarding inventory levels and distribution routes. As a result, the company reduced operational costs by 15% while improving delivery times.
Another example can be found in the healthcare sector, where a hospital implemented an RL-based scheduling system for its operating rooms. This solution learned from past usage patterns and patient needs to allocate resources more effectively. Consequently, the hospital achieved a 25% increase in surgical throughput without the need for additional staff or facilities.
Key Benefits from Successful Implementations:
- Cost Reduction: Real-time optimizations lead to lower operational expenses.
- Efficiency Gains: Streamlined processes enhance productivity.
- Data Utilization: Makes use of existing data to improve decision-making.
These examples demonstrate how RL solutions can significantly transform business operations when applied correctly. They also illustrate the potential ROI that can be achieved through careful implementation and analysis.
Challenges Encountered
Despite the advantages, case studies also shed light on the challenges organizations face when deploying RL solutions. In one instance, a financial services company attempted to integrate a trading algorithm based on RL but encountered issues with data quality. Inaccurate data significantly hindered the algorithm's performance, leading to unexpected losses. The company had to rectify its data collection and validation processes before witnessing any positive results.
Another case involved a retail chain that implemented an RL solution for personalized marketing. While the initial results were promising, the company struggled with integrating the RL model into its existing CRM systems. This disconnection led to implementation delays and required additional resources for synchronization.
Common Challenges in Implementation:
- Data Quality Issues: Poor data can significantly affect performance.
- Integration Difficulties: Ensuring compatibility with existing systems is often complex.
- Resource Intensiveness: Requires time and skilled personnel for effective deployment.
Overall, these challenges highlight the need for thorough preparation and understanding before integrating RL solutions. Learning from past implementations can help new adopters avoid common pitfalls and achieve better outcomes.
User Perspectives on RL Solutions
User perspectives play a crucial role in understanding the effectiveness and practical implications of Reinforcement Learning (RL) solutions in B2B software environments. It is vital to evaluate how users actually experience these systems to ensure they are meeting their operational needs and expectations. By embracing feedback and testimonials from those who use RL solutions, stakeholders can gain insights into both the strengths and weaknesses of these technologies.
The focus on user perspectives not only highlights the practical applications of RL but also enables vendors to refine their offerings. Users often position themselves at the intersection of technical functionality and real-world requirements. Hence, prioritizing their experiences can lead to improved software design and more effective implementations, ultimately benefiting the organizations involved.
User Testimonials
User testimonials offer a direct line of feedback from those interacting with RL solutions firsthand. These accounts provide valuable insights on how effectively these systems contribute to operational efficiency and decision-making processes within their organizations. For instance, many users note an improvement in predictive accuracy which facilitates better resource allocation.
Here are key benefits often mentioned in testimonials:
- Enhanced Efficiency: Users frequently report time savings through automation of repetitive tasks, leading to more focus on strategic initiatives.
- Scalability: Many find that RL solutions adapt well as their business grows, easily accommodating increasing data volumes and user loads.
- User Interface: Positive feedback often highlights intuitive interfaces that simplify complex processes, making it easier for non-technical users to engage with the technology.
However, some users have shared concerns as well. Issues such as lengthy setup times or steep learning curves can impact initial user satisfaction. Addressing these concerns in future developments can foster better user experiences.
Expert Opinions
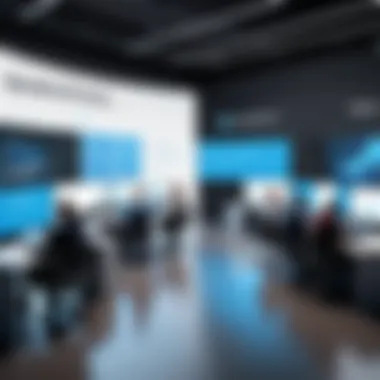
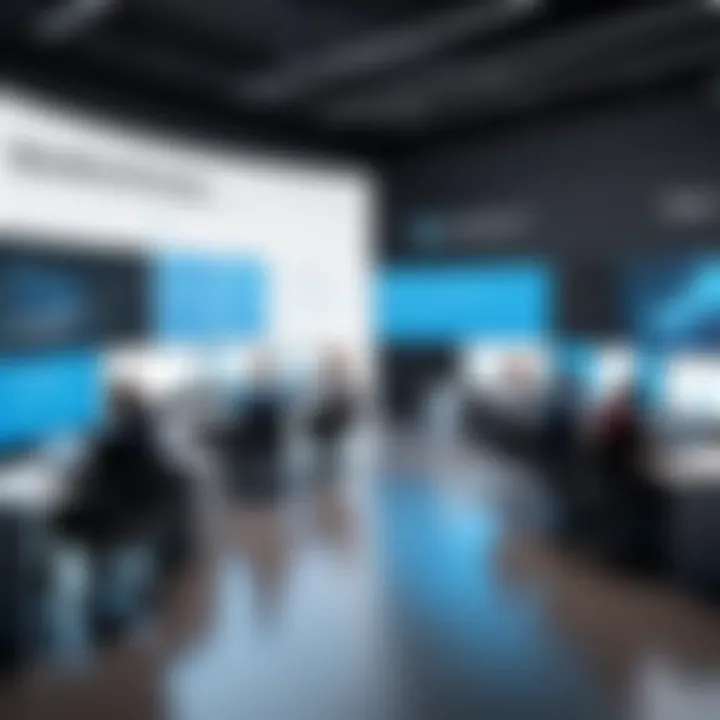
Expert opinions provide a broader perspective on RL solutions, often drawing on comprehensive analyses and industry benchmarks. Experts emphasize key considerations for organizations implementing these solutions. One common area of focus is the importance of robust data input for achieving optimal outcomes.
Experts often discuss:
- Data Quality: High-quality, relevant datasets are essential for training effective RL algorithms. Poor data practices can lead to suboptimal performance, which, in turn, affects user satisfaction.
- Integration Challenges: Many experts underline the need for smooth integration of RL solutions with existing business systems. Failure to do so can lead to disruptions and hinder adoption.
- Ethical Considerations: As RL solutions become more pervasive, experts advocate for ethical frameworks guiding their application to prevent biases and ensure fairness in business decisions.
In summary, gathering user testimonials and expert insights contributes significantly to the understanding of RL solutions in B2B software. By integrating these perspectives, organizations can enhance decision-making and operational efficiency, aligning their technological strategies with real-world needs and expert recommendations.
Future Trends of RL Solutions
The landscape of reinforcement learning (RL) solutions in B2B software is rapidly evolving. Understanding future trends is crucial for businesses aiming to leverage these technologies for competitive advantage. As RL matures and integrates more deeply into enterprise systems, anticipating upcoming innovations allows organizations to stay ahead in operational efficiency, customer satisfaction, and strategic growth.
Technological Advancements
With advancements in artificial intelligence and data analytics, the capabilities of RL solutions are expanding.
- Deep Reinforcement Learning: This method combines neural networks with RL principles. It enhances the algorithms’ performance in more complex environments. Companies are using this to optimize logistics, customer interactions, and resource management.
- Transfer Learning: This allows RL models to apply knowledge gained in one context to another, reducing training time and improving adaptability. For example, a model trained in retail environments can utilize its experience in finance.
- Real-Time Data Processing: Improvements in cloud technology enable RL solutions to analyze data in real-time. This capability allows for quicker decision-making and a more responsive business model.
Embracing these technological advancements can substantially enhance an organization’s learning and adaptation strategies. As a result, companies can more effectively respond to market fluctuations and consumer behavior shifts.
Market Forecast
The market for RL solutions in B2B software is projected to experience significant growth in the coming years. Analysts suggest that the compound annual growth rate (CAGR) will exceed 25% over the next five years. Some contributing factors include:
- Increased Adoption: More businesses are recognizing the value of RL in automating and optimizing processes. Enterprises in both manufacturing and service sectors are implementing RL-driven tools for efficiency gains.
- Growing Demand for Personalization: B2B companies focus more on personalized customer experiences. RL solutions offer insights that drive tailored offerings, enhancing customer loyalty and retention.
- Investment in AI Technologies: Significant capital is being directed into AI and machine learning research. This investment fuels RL innovation, creating more robust and scalable solutions that fulfill diverse business needs.
"The next decade will witness a seismic shift in how businesses approach operational strategies with RL at the core."
These forecasts suggest that organizations which invest in RL capabilities now are likely to reap long-term rewards, establishing themselves as leaders in their respective industries.
Implementing RL Solutions Effectively
Implementing RL solutions in B2B environments requires a detailed and strategic approach. Such solutions can significantly enhance operational efficiency and decision-making processes within organizations. However, the effectiveness of these implementations greatly relies on understanding both the technology and the organizational context in which it operates. Gaining clarity about internal workflows, existing systems, and team dynamics is vital. This section highlights best practices and outlines essential change management strategies that can facilitate a smooth adoption of RL systems.
Best Practices
To maximize the benefits of RL solutions in your organization, consider the following best practices:
- Define Clear Objectives: Establish the specific goals you wish to achieve with the implementation. This could range from improving efficiency in existing processes to scaling operations more effectively.
- Engage Stakeholders Early: Identify and involve key stakeholders in the planning phase. Engaging users from diverse departments can help to uncover unique insights and foster a sense of ownership towards the new solution.
- Choose the Right Software: Not all RL solutions fit every organization. Assess various options and evaluate their compatibility with your existing IT infrastructure and business processes.
- Pilot Programs: Start with a pilot implementation to troubleshoot and refine the process. This allows for adjustments based on user feedback before a broader rollout.
- Training and Support: Invest in comprehensive training programs to equip team members with the necessary skills to leverage the new solution effectively. Continuous support mechanisms are also vital to assist users in overcoming any challenges they may encounter.
By adhering to these best practices, businesses can streamline the implementation process and lay a solid foundation for realizing the full potential of RL solutions.
Change Management Strategies
Change management is a critical aspect of the successful implementation of RL solutions. Organizations often face resistance when altering existing workflows or adopting new technologies. Here are key strategies to manage this transition:
- Communicate Clearly: Transparency throughout the implementation process builds trust. Keep all stakeholders informed about reasons for the change, expected outcomes, and any potential disruptions.
- Create a Change Champions Network: Identify and empower individuals within the organization who can advocate for the change. Their enthusiasm can help influence others to embrace the new system.
- Monitor Progress and Gather Feedback: Regularly assess how the implementation is progressing. Collect feedback from users and make necessary adjustments based on their experiences.
- Reinforce the Changes: After implementation, continue to promote the benefits observed because of the RL solution. Regular updates about wins and successful cases will help in solidifying the new practices.
Implementing RL solutions effectively is not just about technology; it is about the people and processes that surround it. A focus on both the technical aspects and the human element will yield a smoother transition and ultimately better outcomes.
"Successful implementation of RL solutions is a careful balance of technical proficiency and change management finesse."
By strategically approaching both best practices and change management, organizations can better position themselves for success with RL solutions.
The End
In this article, we explored the role of RL solutions within the B2B software landscape. The conclusion draws together the vital insights gained from the discussions throughout the piece. Understanding RL solutions is essential for any organization looking to improve its operational efficiency and drive strategic growth.
Summary of Findings
The research indicates that RL solutions can drastically enhance decision-making processes within businesses. They empower organizations by providing data-driven insights and personalized recommendations. Key findings include:
- Increased Operational Efficiency: Businesses utilizing RL solutions often report faster and more accurate decision-making capabilities.
- Enhanced User Experience: Tailored solutions lead to higher user satisfaction, promoting client retention and loyalty.
- Cost Reduction: Efficient processes translate into cost savings, which is a critical aspect for small to medium enterprises.
By synthesizing these factors, it becomes clear that RL solutions hold a pivotal role in shaping modern business practices. This shift towards data-centric decision-making influences not only how companies operate internally but also how they position themselves in the market.
Final Thoughts
As B2B environments continue to evolve, embracing RL solutions is no longer optional but a necessity for forward-thinking enterprises. Organizations should focus on assessing their unique needs and challenges when considering implementation.
"Investing in RL solutions is akin to equipping your business with a sophisticated analytical brain that continuously learns and adapts."
In summary, the right RL tools can transform a business into a more agile, efficient, and competitive entity. Future research and developments in this field will likely reveal even more advantages and applications, encouraging wider adoption across various sectors. The ultimate goal should be clear—leverage the power of RL solutions to create sustainable growth and operational excellence.