Mastering VMO A/B Testing for Marketing Success
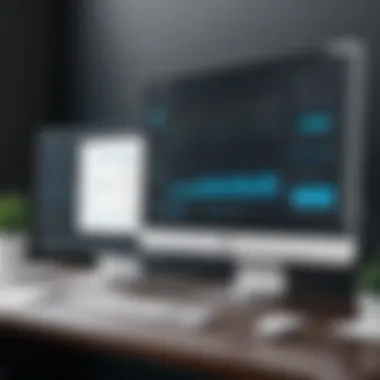
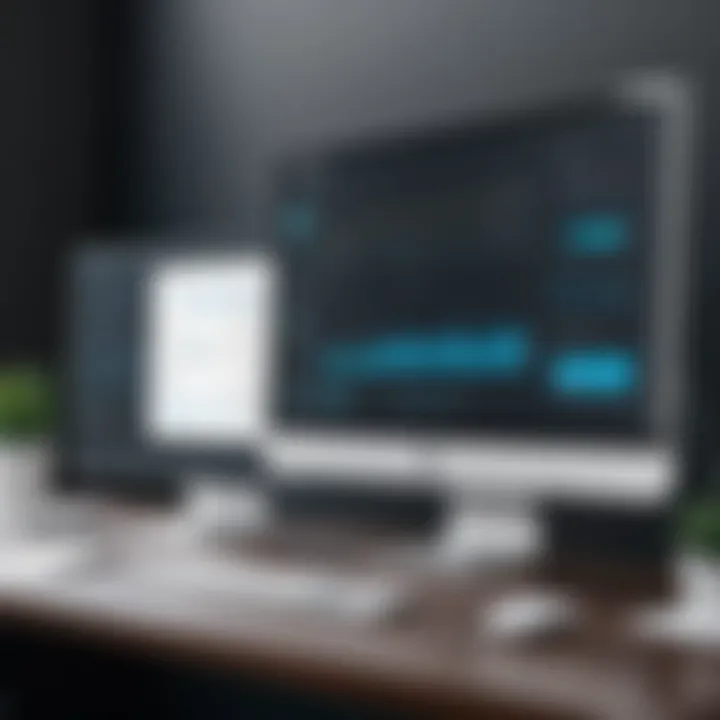
Intro
In the rapidly evolving landscape of digital marketing, Value Management Organizations (VMOs) rely heavily on strategies that leverage data-driven insights. Among these strategies, A/B testing stands out as a powerful method to optimize marketing efforts and enhance product performance. Understanding the nuances of VMO A/B testing is essential for decision-makers who aim to utilize this tool effectively.
This article will unravel the complexities of VMO A/B testing, examining its importance in shaping marketing strategies, the methodology behind it, and the best practices for successful implementation. By incorporating case studies and expert analysis, readers will acquire a comprehensive understanding that aids in strategic decision-making.
Software Overview
Software Description
VMO A/B testing software facilitates the comparison of two or more variations of a marketing asset, be it an email, ad campaign, or webpage. By systematically analyzing user interaction with these variations, it determines which one performs better in terms of key performance indicators, such as conversion rates or click-through rates. This process is critical for VMOs focused on performance optimization.
Key Features
The features of A/B testing software are instrumental for organizations aiming to refine their strategies. Common characteristics include:
- User Segmentation: Ability to segment audiences for targeted testing.
- Real-Time Analytics: Instant feedback on performance metrics.
- Multivariate Testing: Support for testing several variables at once.
- Integrations: Compatibility with existing marketing tools.
- Reporting Tools: Comprehensive reports that outline successes and areas for improvement.
These features empower VMOs to make informed decisions based on actionable data.
Pricing Models
Subscription Options
Many VMO A/B testing platforms offer subscription-based pricing, allowing organizations to choose a plan that suits their needs. Common tiered options include:
- Basic Plan: Ideal for startups or small businesses, offering limited features.
- Professional Plan: Designed for medium-sized organizations with advanced features and analytics.
- Enterprise Plan: Tailored for large corporations requiring robust testing capabilities and dedicated support.
One-Time Purchase
Alternatively, some software developers offer a one-time purchase option. This model can be appealing for organizations looking to make a long-term investment without ongoing costs. However, it is essential to consider the potential need for updates and support.
"Successful A/B testing is not just about finding the winner; it's about understanding user behavior and preferences."
Prelims to VMO A/B Testing
VMO A/B testing is a critical aspect of optimizing marketing strategies in modern business environments. It enables organizations to make informed decisions based on empirical data rather than intuition. The ability to test different versions of a product, service, or marketing strategy against each other allows companies to determine the most effective approach to achieve desired outcomes.
A/B testing involves comparing two variations, A and B, to measure their performance across certain metrics. This method is especially valuable in the B2B landscape, where the stakes are often high, and decision-makers need clear evidence to justify their choices.
When organizations leverage VMO A/B testing, they benefit in several ways:
- Improved understanding of customer preferences and behaviors.
- Enhanced product performance through iterative testing.
- More effective marketing strategies based on actual user data.
- Reduced risk when launching new initiatives by validating concepts first.
Such advantages underscore the importance of this testing method in refining approaches for better alignment with market demands.
Defining VMO A/B Testing
VMO A/B testing stands for Value Management Objectives A/B Testing. It is a systematic approach where variations of a single element are tested to see which one yields better performance regarding specific objectives. In VMO testing, the focus is not just on what performs well, but also on how well it aligns with strategic value management goals.
A key feature of this testing approach lies in its ability to not only measure performance but also to gauge the impact of those changes on broader organizational goals. This could mean evaluating the conversion rate of a landing page or the effectiveness of a sales email.
The Relevance of A/B Testing in the B2B Landscape
In the B2B sector, A/B testing is more than just a useful tool; it is often a necessity. Businesses face unique challenges and complexities. Decisions can involve substantial financial resources, making it vital to minimize risks. Consequently, A/B testing helps in balancing these risks with the potential rewards of new implementations.
The relevance of A/B testing in B2B can be highlighted through several aspects:
- Data-Driven Decisions: B2B entities often deal with long sales cycles and multiple stakeholders. A/B testing provides data to support decisions, ensuring that choices are made based on evidence.
- Market Segmentation: Each business has different market segments. A/B testing allows companies to tailor their strategies to different segments, improving engagement and effectiveness.
- Enhanced ROI: By testing initiatives prior to full-scale rollout, organizations can focus their investments on strategies that have already proven effective, ultimately leading to better returns.
In summary, understanding VMO A/B Testing lays the foundation for effective strategic decision-making in B2B environments. The results can directly influence numerous aspects of the business, from product development to marketing campaigns.
Core Principles of A/B Testing
A/B testing is a foundational method that enhances decision-making, particularly in a B2B context. Its importance cannot be understated, as it allows organizations to make informed choices based on data rather than intuition. The core principles address the fundamental aspects necessary for conducting effective A/B tests and obtaining reliable results. These principles ensure that decision-makers can translate insights into practical actions to improve marketing strategies and optimize product performance.
Understanding Hypothesis Development
A hypothesis is the initial statement that places a claim to be tested. Developing a strong hypothesis is essential in guiding the testing process. A well-crafted hypothesis provides clarity on what is being tested and the expected outcome. In the context of VMO A/B testing, this means formulating a statement that links an action to a measurable result.
For example, an organization might hypothesize that changing the wording of a button from "Buy Now" to "Get Started" will increase click-through rates by at least 10%. This statement presents a clear expectation, and by testing it, the company can ascertain whether this change resulted in the anticipated behavior.

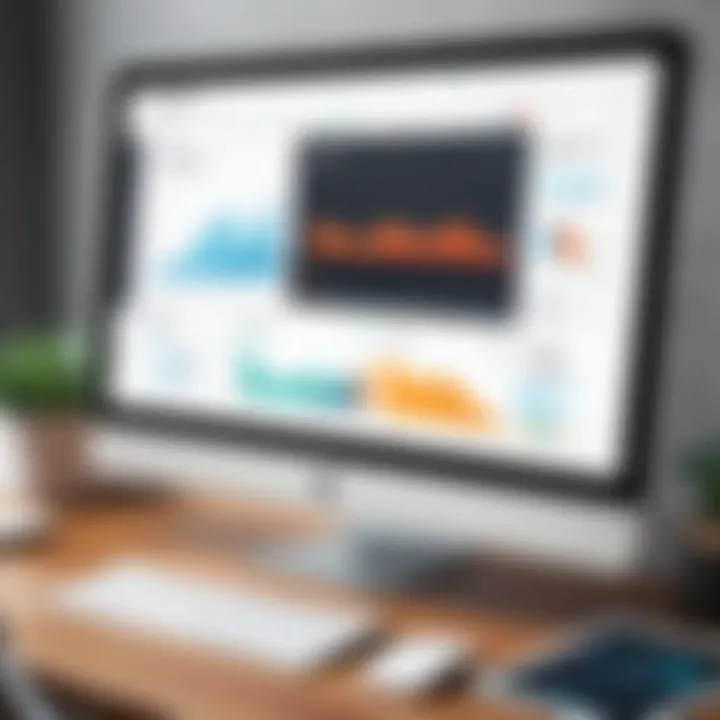
Key considerations for hypothesis development include:
- Clear Objective: Ensure that the hypothesis addresses a specific business goal.
- Measurable Outcome: Frame it so that success can be quantitatively assessed.
- Testable Approach: Ensure that the hypothesis can be explored through an experiment.
By maintaining a structured approach to hypothesis development, organizations can embark on their testing journey with a focus that leads to actionable insights.
Establishing Clear Metrics for Success
Metrics are the backbone of any testing initiative. Establishing clear metrics for success is critical in measuring the effectiveness of your A/B tests. Without clearly defined metrics, it becomes challenging to determine whether a test succeeded or failed.
Common metrics to consider include conversion rates, engagement levels, and user retention rates. When deciding on metrics, it is crucial to align them with business objectives. For instance:
- Conversion Rate: The percentage of visitors who complete a desired action such as signing up for a newsletter.
- Engagement Levels: This could include time spent on a website or number of pages viewed per visit.
- User Retention: Measuring how many users return after their initial visit.
"Establishing robust metrics not only guides the testing process but also provides a framework for continuous improvement."
The clarity of these metrics allows for straightforward analysis and enables decisions about next steps based on the outcome of the tests.
In summary, the core principles of A/B testing—effective hypothesis development and clear metric establishment—are essential for successful experimentation. These principles help ensure that organizations are set on a path toward data-informed decision-making.
The VMO Approach to A/B Testing
The VMO (Value Management Organization) approach to A/B testing is a critical framework that enables organizations to effectively assess and enhance their marketing strategies. As businesses strive to derive more value from their operations, integrating a value management perspective with A/B testing becomes essential. This section will delve into two key aspects: integrating value management with A/B testing, and identifying key performance indicators.
Integrating Value Management with A/B Testing
Value management in a business context refers to the process of understanding, optimizing, and maximizing the value delivered to customers. When integrated with A/B testing, this approach focuses on aligning testing strategies with the overall value objectives of the organization. This ensures that the testing efforts are not only rigorous but also relevant to business goals.
One of the main benefits of this integration is the clarity it provides in the decision-making process. Organizations can map out the customer journey and identify which aspects of their offerings need testing to drive value. It promotes a more structured approach by tailoring experiments to address specific value-related questions.
Moreover, this integration fosters a culture of continuous improvement. By frequently testing ideas against customer expectations, companies can make data-driven changes rather than relying solely on assumptions. This approach ensures that the organization responds promptly to market trends, which can lead to better customer satisfaction and retention rates.
Identifying Key Performance Indicators
Key Performance Indicators (KPIs) serve as measurable values that indicate how effectively a company is reaching its business objectives. In the context of VMO A/B testing, identifying the right KPIs is paramount. The right KPIs provide insight into whether a test is successful or not and help prioritize future tests based on potential value gain.
Selecting meaningful KPIs involves several considerations:
- Relevance to Goals: Each KPI should directly correlate with the strategic objectives of the organization. This alignment ensures that every test conducted provides actionable insights.
- Measurable: The indicators should be quantifiable. This allows easy tracking over time.
- Actionable: KPIs must guide decision-making. If a metric indicates poor performance, it should lead to concrete actions for improvements.
Common KPIs for A/B testing include conversion rates, average order values, and engagement rates, among others. Businesses should focus on KPIs that truly reflect the value being delivered to customers, promoting a focus not just on the outcome of tests, but on the broader impacts on the organization’s value delivery.
Important Note: Aligning testing metrics with value delivery can significantly improve insight and decision-making in organizations.
In summary, the VMO approach to A/B testing offers a structured method for enhancing marketing strategies. By integrating value management principles and clearly identifying KPIs, organizations can leverage A/B testing to drive significant improvements and maximize customer value.
Designing A/B Tests Effectively
Designing A/B tests effectively is a cornerstone of the scientific approach to marketing and product optimization. In the realm of VMO A/B testing, a well-structured design can significantly influence the results and actionable insights derived from those tests. The effectiveness of A/B testing goes beyond mere comparison; it encapsulates the art and science of choosing the right elements to test, while ensuring the outcomes can be reliably interpreted. Organizations that invest time in designing their tests can expect to gain clearer insights, foster innovation, and ultimately drive better performance in their strategies.
Selecting Variables for Testing
When it comes to selecting variables for testing, careful consideration is vital. The first step is identifying which elements of a marketing strategy or product interface are most likely to impact user behavior. This could range from user interface design on a website to the language used in a marketing email. Key variables often include:
- Headlines and Content: Changing the headline can influence click-through rates significantly.
- Call to Action (CTA): The formulation of the CTA button, including its color, text, and placement, might lead to different conversion rates.
- Images and Visuals: Presenting different images or graphics can affect user engagement and perception.
Testing different variables allows organizations to isolate the effects of these modifications on user behavior. However, it's crucial to restrict the number of variables tested at once to maintain clarity in results. Experimenting with too many changes can lead to confusion about which variable caused any observed shift in user engagement.
"The success of A/B testing relies upon the clarity and focus of your variables selected for testing."
Sample Size Considerations
Determining the sample size for A/B testing is critical for the validity of the test outcomes. A larger sample size generally leads to more reliable results, but it also comes with increased time and resource allocation. Factors to consider when deciding the sample size include:
- Statistical Significance: The goal should be to attain a sample size that ensures any outcome is statistically significant. A common benchmark is to achieve a confidence level of 95%.
- Projected Conversion Rate: Understanding the expected baseline conversion rate helps in calculating the necessary sample size. For example, a site with a 2% conversion rate will need more participants to detect a meaningful difference compared to a site with a higher conversion rate.
- Minimum Detectable Effect (MDE): This refers to the smallest change in conversion rate that is practical to detect. A smaller MDE would generally require a larger sample size.
Thus, balancing between sample size, resource allocation, and desired precision can significantly enhance the testing process. Organizations should also consider the duration of their tests, ensuring sufficient time for all variations to be evaluated under similar conditions and to account for any external variables that might skew results.
Executing A/B Tests: Best Practices
Executing A/B tests with effective practices is crucial for generating reliable and actionable results. Best practices ensure that the tests are not only statistically valid but also offer insights that can guide decision making in a business environment. Proper execution avoids common errors and increases the likelihood of uncovering valuable insights that can enhance marketing strategies.
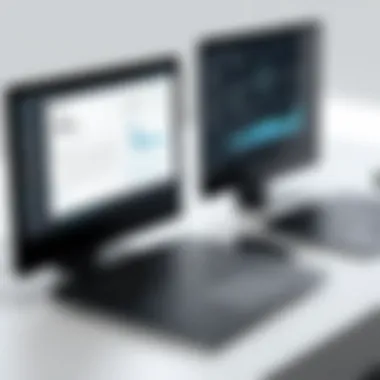
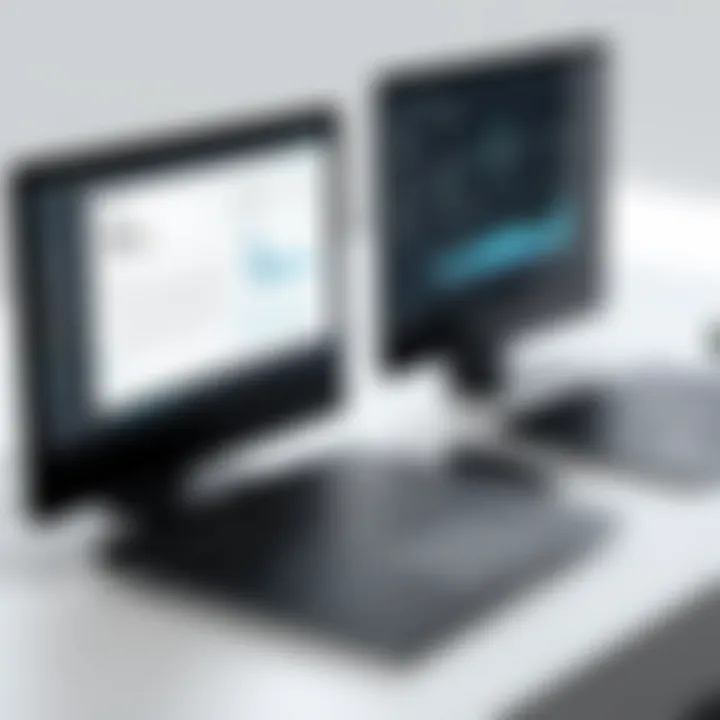
Here are key elements to consider:
- Reliability of Results: Following best practices helps maintain the integrity of the results, making them more reliable.
- Use of Resources: Efficient execution maximizes resource utilization. This is important for businesses looking to optimize costs.
- Ease of Interpretation: A structured approach simplifies the interpretation of test results, enabling quicker decision-making.
By adhering to best practices, businesses can effectively leverage A/B testing as a strategic tool.
Test Duration and Timing
Test duration plays a critical role in A/B testing. A well-calculated duration ensures that the results are representative of user behavior across different times and scenarios. If the duration is too short, variances due to external factors may influence outcomes. Conversely, overly long tests can be resource-intensive without yielding new insights.
Consider the following when determining test duration:
- Traffic Volume: Higher traffic can shorten the required duration as results stabilize faster.
- Seasonal Trends: Running tests during holiday periods or special events might skew results.
- Statistical Significance: Ensure that the sample size achieves statistical significance before concluding the test.
Optimal timing can significantly affect the outcomes. Timing should consider factors such as industry patterns and consumer behavior.
Randomization Techniques
Randomization is fundamental in A/B testing to eliminate bias. This technique ensures that each participant has an equal chance of being assigned to any variant, which enhances the credibility of the results.
Common randomization methods include:
- Simple Random Sampling: Assigns participants randomly without any criteria. This method is straightforward but can be less effective in segmented or targeted campaigns.
- Stratified Random Sampling: Ensures that specific subgroups are adequately represented in the test. This is useful when contrasting different user profiles.
- Dynamic Allocation: Adjusts the allocation of users in real-time based on incoming data. This post-administration modification can enhance the test's efficiency.
By employing rigorous randomization techniques, businesses can mitigate biases and uncover genuine user preferences.
"Randomization is key to isolating the true effect of your changes.”
Analyzing A/B Test Results
Analyzing the results of A/B tests is crucial in leveraging the insights gained from various experiments. The core objective of A/B testing is to determine which version of a given element performs better. However, without proper analysis, the data collected can lead to misinterpretations. In the context of VMO A/B testing, this becomes essential for informed decision-making. The implications of findings go beyond numerical improvements; they can signal the direction of future strategies as well. By effectively interpreting results, one can optimize marketing strategies and enhance product offerings, all while measuring against set goals.
Statistical Methods for Interpretation
Statistical methods serve as the backbone for data interpretation in A/B testing. They allow for the testing of hypotheses and understanding the significance of observed differences. Common statistical approaches include:
- t-tests: This tests whether two groups have significantly different means. In A/B tests, it can identify if the variation in performance metrics between two groups is statistically significant.
- Chi-square tests: Used for categorical data to examine the association between two variables. This is important when analyzing actions such as clicks or purchases in A/B testing.
- Confidence intervals: These provide a range of values, indicating the reliability of results. A confidence interval can help assess whether a result falls within an acceptable likelihood of success.
Using these methods appropriately is vital. They ensure that conclusions drawn are based on sound scientific principles. Implementing statistical significance thresholds allows businesses to determine the likelihood that results occurred by chance.
"Proper statistical analysis transforms raw data into actionable insights. Without it, you navigate blindly."
Common Pitfalls in Data Analysis
While analyzing A/B test results, it is crucial to be aware of frequent mistakes that can lead to inaccurate interpretations. Here are some of the common pitfalls to avoid:
- P-hacking: This occurs when a researcher manipulates data until statistically significant results appear. This can lead to confirmation bias.
- Ignoring sample size: A small sample size can produce unreliable results. Ensure that the sample is large enough to represent the target population.
- Overlooking confounding variables: Failing to account for other variables affecting outcomes can skew results. Analyzing the data without understanding all influencing factors can lead to false conclusions.
- Inadequate tracking: If metrics are not tracked precisely, you may not capture the full story. Set up tracking mechanisms before the tests begin to ensure comprehensive data collection.
Understanding and avoiding these pitfalls enables more robust analysis, leading to better decisions supported by empirical evidence. This strengthens overall marketing strategies and helps organizations harness the true potential of their A/B testing efforts.
Case Studies in VMO A/B Testing
Case studies are critical in demonstrating the practical applications of VMO A/B testing. They serve not only as examples of successful executions but also highlight the lessons that can be learned from failures. This section will examine important elements such as benefits, considerations, and the overall relevance of these case studies in advancing one's understanding of VMO A/B testing.
Successful Implementations
Successful A/B testing implementations can provide a roadmap for organizations looking to optimize their marketing strategies. One notable case is that of a leading technology firm, Tech Innovations Inc. They undertook a comprehensive A/B test to analyze the effectiveness of their email marketing campaigns. They created two versions of an email that differed only in subject lines.
- Version A: "Unlock the Future of Technology Today"
- Version B: "Discover Innovative Solutions for Your Business"
Through careful data collection and analysis, Tech Innovations Inc. found that Version B outperformed Version A by a significant margin, leading to a 25% increase in open rates and a 15% boost in click-through rates. This positive outcome not only improved their marketing efforts but also reinforced the significance of targeted messaging, prompting them to further tailor their communications.
Additionally, their commitment to continual testing enhanced their understanding of customer behavior. By implementing VMO principles, they could attach value management to their testing, ensuring each campaign aligned with their strategic objectives.
Lessons Learned from Failures
Not every A/B test leads to successful outcomes. Sometimes, valuable lessons arise from failures, which are equally informative. A case that exemplifies this is an online retail company, Fashion Trends. They conducted an A/B test to evaluate two layouts for their product page.
- Layout A: Featured a grid view format.
- Layout B: Used a list view format with larger images.
Expecting Layout B to proliferate engagement due to a cleaner aesthetic, they were surprised to conclude that Layout A significantly drove higher conversion rates. The unexpected outcome pointed to several factors that contributed to the results:
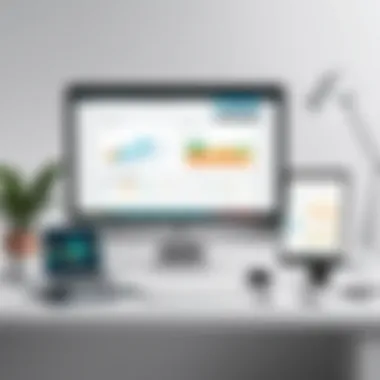
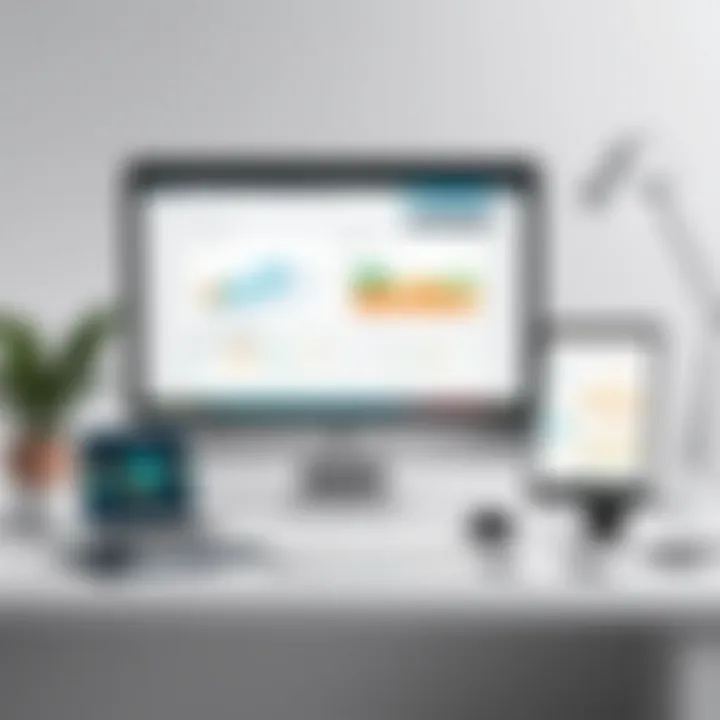
- Users found the grid layout more intuitive, leading to better navigation.
- The removal of distractions in Layout A kept the focus on the product images.
- Feedback from user surveys highlighted that familiarity in layout influenced user comfort.
These missteps taught Fashion Trends to avoid assumptions based solely on aesthetics. They learned the importance of using data-driven decision-making and investing in user feedback.
Challenges in A/B Testing
In the realm of VMO A/B testing, grasping the challenges is as crucial as understanding its mechanisms. A/B testing can be powerful, yet fraught with complexity. Properly addressing these challenges can significantly enhance the validity of results and the ultimate success of marketing strategies.
Managing Bias and External Variables
Bias can skew A/B testing outcomes and lead to misleading conclusions. It is essential to recognize various forms of bias that can occur. Selection bias arises when the sample used in testing is not representative of the larger population. For instance, if only a specific segment of users, perhaps those more engaged, are tested, the findings may not reflect what all users would experience. Additionally, confirmation bias can occur when teams unintentionally favor outcomes that support pre-existing beliefs while ignoring data that contradicts those ideas.
To counter these biases:
- Randomization is key. Ensure that test groups are randomly assigned. This helps mitigate selection bias.
- Blinding can also reduce confirmation bias. For instance, ensuring data analyzers do not know which variant they are evaluating can provide a more objective view.
- Establish clear hypotheses before testing. A well-defined hypothesis guides decision-making during analysis.
Another concern is external variables that can influence results. These variables include changes in consumer behavior, seasonal trends, or even marketing campaigns coinciding with the A/B test period. Recognizing and controlling for these variables is essential. Using statistical models can help isolate the true impact of changes made. It is also worthwhile to conduct tests over a sufficiently long period to capture fluctuations in data.
Consideration: Always account for factors affecting user interaction during testing phases.
Scaling A/B Tests Across Multiple Platforms
Scaling A/B tests is an arduous task, especially for organizations working across various platforms. Each platform may have different user interfaces, behaviors, and audience demographics. Thus, what works on one platform may not yield similar results on another.
Some critical considerations when scaling A/B tests include:
- Platform Consistency: Strive for consistent user experiences across all platforms. Any significant deviation might lead to misleading data.
- Data Integration: Establish robust mechanisms to pull data from different platforms into a central analysis hub. This aids in comprehensive comparison and understanding of results.
- Testing Tools: Utilize tools that support multi-platform A/B testing, like Optimizely or Google Optimize. These tools can streamline the process and provide insights across different environments.
- Feedback Loops: Create systems for continuous feedback from tests. Scale based on insights rather than merely replicating tests across channels.
To conclude, while A/B testing provides valuable insights, challenges such as bias, external variables, and scaling efforts can complicate the process. By proactively managing these elements, organizations can derive stronger conclusions and ultimately refine their marketing strategies.
The Future of A/B Testing in B2B
A/B testing continues to evolve and play a critical role in the B2B landscape. As digital marketing tactics grow more sophisticated, so too must the methods we employ to measure their effectiveness. The future of A/B testing is not just about running tests to determine which variations perform better; it involves a deeper understanding of how emerging technologies can be harnessed to enhance testing processes.
With the rise of Artificial Intelligence and machine learning, businesses can expect more insightful data analyses. These technologies offer predictive modeling capabilities that can identify trends before they become evident through traditional A/B testing methods. By adopting these innovations, B2B organizations can gain a competitive edge, allowing for more agile decision-making and refined marketing strategies.
In this dynamic environment, A/B testing must also adapt to new consumer behaviors. As data privacy concerns rise and regulations tighten, understanding how to conduct A/B tests ethically and transparently will become paramount.
"The future of A/B testing hinges on the seamless integration of evolving technologies and consumer insights."
Emerging Technologies and Innovations
Technological advancements are reshaping how A/B testing is conducted. For instance, the utilization of AI algorithms can automate the testing process, making it faster and more efficient. AI can predict not only performance outcomes but also which variations are likely to engage consumers more effectively. This predictive analysis can save significant time and resources while increasing accuracy in results.
Moreover, tools that integrate seamlessly with platforms like HubSpot and Google Analytics can offer real-time optimization suggestions. These technologies allow marketers to make data-driven adjustments during the testing phase rather than waiting until the end of the test.
- AI-Powered Tools: Tools such as Optimizely and VWO leverage AI for enhanced data modeling.
- Integration with Analytics: Solutions that automatically sync A/B testing data with business intelligence platforms provide comprehensive insights.
- Automated Test Management: Emerging platforms help manage multiple tests across various customer touchpoints, reducing manual oversight.
By embracing these technological innovations, B2B marketers can create more personalized experiences and increase conversion rates.
Evolving Consumer Behavior Trends
Consumer behavior is shifting at an unprecedented pace. As B2B buyers become younger and more tech-savvy, their expectations for personalized experiences intensify. They prefer brands that understand them and tailor messages to their unique needs. A/B testing must, therefore, evolve with these changing expectations.
A/B testing will need to address not only what converts consumers but also why certain variations resonate with them. This deeper understanding of motives and preferences can lead to more effective marketing strategies tailored to specific segments.
- Increased Demand for Personalization: Marketers need to leverage A/B tests to discover what personalized content performs best.
- Cross-Device Testing Importance: Consumers interact with brands on various devices; A/B testing should reflect performance on mobile, desktop, and other platforms.
- Ethical Marketing Practices: As consumers grow wary of data usage, ethical considerations in A/B testing will gain importance. Transparency will foster trust, which is vital for maintaining brand loyalty.
In summary, as A/B testing continues to adapt to new technologies and shifts in consumer behavior, it will become an indispensable tool for B2B organizations aiming to maintain relevance and drive success in their marketing efforts.
The End and Recommendations
Concluding an exploration into VMO A/B testing entails summarizing the critical insights derived from the methodology and its implementation in business contexts. This part of the article is essential, as it encapsulates the core learnings that readers must retain. Specifically, it focuses on emphasizing the importance of structured testing and continuous improvement. It urges organizations to utilize data-driven decisions to hone their marketing strategies and product development.
Summarizing Key Takeaways
In summary, several key aspects emerge from our discussions around VMO A/B testing:
- The Importance of Hypothesis: Creating a solid hypothesis lays the foundation for meaningful tests. Without this, results may lead to misguided conclusions.
- Metrics Matter: Establishing clear, measurable indicators of success is vital. Metrics like conversion rates and user engagement provide a quantifiable way to assess outcomes.
- Execution is Key: Proper test execution, including randomization and duration, can significantly influence results. Attention to these details prevents biases from skewing data.
- Data Analysis is Critical: Understanding how to interpret data using statistical methods is crucial for drawing valid conclusions. Organizations need to focus on avoiding common analytical pitfalls.
- Learning from Case Studies: Analyzing previous real-world cases supports learning. Both successes and failures yield valuable lessons that organizations can leverage for future tests and strategies.
"Data never sleeps, and neither should your insights from it."
Next Steps for Implementation
Implementing VMO A/B testing effectively involves several next steps:
- Develop a Robust Testing Framework: Define the scope of your tests clearly. This includes mapping out what will be tested, how results will be measured, and determining success criteria.
- Invest in Analytics Tools: Utilize analytical tools that can track user interactions efficiently. Tools like Google Analytics, Mixpanel, or Kissmetrics can enhance your ability to gather insights.
- Train Team Members: Ensure that key members of your team understand A/B testing principles and techniques. Consider workshops or online courses that emphasize statistical analysis and test structuring.
- Start Small: Begin with small tests to build a foundational understanding. Use findings from smaller experiments to inform larger testing strategies.
- Iterate Based on Data: Build a culture of continual learning and adaptation. Use insights gained from A/B testing to refine strategies, ensuring that your organization remains agile and responsive to data-driven insights.
By following these steps, organizations can unlock the full potential of VMO A/B testing, leveraging it not just for immediate gains but also for long-term strategic advantages.